A rolling bearing fault diagnosis method based on IAGA-SVM
A rolling bearing and fault diagnosis technology, which is applied in mechanical bearing testing, gene models, special data processing applications, etc., can solve problems such as low classification accuracy and low efficiency, and achieve clear and accurate expression, optimal classification and prediction effects, and fault diagnosis Reasonable and effective effect of the model
- Summary
- Abstract
- Description
- Claims
- Application Information
AI Technical Summary
Problems solved by technology
Method used
Image
Examples
Embodiment 1
[0053] Embodiment 1: as figure 1 As shown, a rolling bearing fault diagnosis method based on IAGA-SVM first adopts the method of wavelet change to extract the features of the rolling bearing fault data, and normalizes them to form training samples and train to obtain the SVM model; then use the improved The adaptive genetic algorithm optimizes the penalty factor C and the kernel function parameter γ of the SVM model to obtain the optimized SVM model, namely the SVM fault diagnosis model, and finally uses the SVM fault diagnosis model to diagnose the rolling bearing fault.
[0054] Because the process of data collection often contains inevitable noise and invalid information, if the original data is used without processing, the result will always be unsatisfactory. Therefore, in order to obtain better experimental results, the present invention uses wavelet transform to perform feature extraction on the original signal after denoising, so as to obtain effective information in t...
Embodiment 2
[0091] Embodiment 2: In this embodiment, specific rolling bearing fault signals are extracted, specifically, wavelet packets are selected to perform feature extraction on denoised signals. The 3-layer decomposition of the wavelet packet will obtain 8 frequency bands, and then reconstruct the signal, and the normalized energy values of each frequency band form a feature vector. The energy of each frequency band in the four states is shown in Table 1:
[0092] Table 1 Energy table of each frequency band in four states
[0093]
[0094]
[0095] After normalization, figure 2 The energy distribution of each frequency band in the four states is intuitively expressed.
[0096] From figure 2 It can be seen that the energy distribution diagrams corresponding to the four states of rolling bearings are quite different, and this difference in energy distribution is very helpful for the identification of fault types.
[0097] Among them, the reconstruction signals of the eig...
PUM
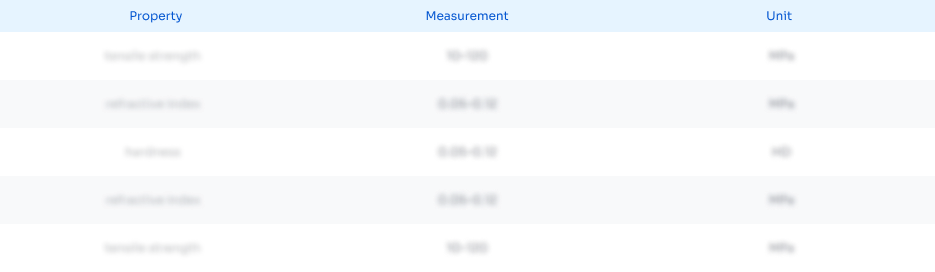
Abstract
Description
Claims
Application Information
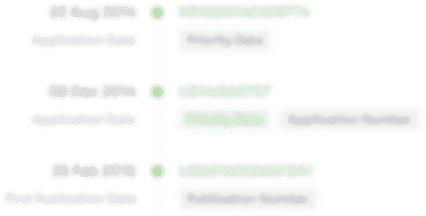
- R&D Engineer
- R&D Manager
- IP Professional
- Industry Leading Data Capabilities
- Powerful AI technology
- Patent DNA Extraction
Browse by: Latest US Patents, China's latest patents, Technical Efficacy Thesaurus, Application Domain, Technology Topic, Popular Technical Reports.
© 2024 PatSnap. All rights reserved.Legal|Privacy policy|Modern Slavery Act Transparency Statement|Sitemap|About US| Contact US: help@patsnap.com