Mixed domain feature bearing fault diagnosis method based on Pearson's correlation coefficient
A Pearson correlation and fault diagnosis technology, which is applied in the testing of mechanical components, the identification of patterns in signals, and the testing of machine/structural components. , to achieve the effect of improving diagnostic accuracy, clear and accurate expression, and improved diagnostic accuracy.
- Summary
- Abstract
- Description
- Claims
- Application Information
AI Technical Summary
Problems solved by technology
Method used
Image
Examples
Embodiment 1
[0120] Embodiment 1: as Figure 1-10 As shown, a mixed-domain characteristic bearing fault diagnosis method based on Pearson correlation coefficient includes the following steps: First, extract 6 time-domain dimensionless vectors, 10 time-domain dimensioned vectors, and 4 frequency-domain vectors from the original signal The eigenvectors, 6 wavelet transform eigenvectors and 10 adaptive noise CEEMDAN eigenvectors of complete integrated empirical mode decomposition, combined with the extracted feature parameters, construct the bearing fault mixed domain feature set; secondly, use the Pearson correlation coefficient to extract Correlation analysis is performed on the mixed domain features of the high-dimensional fault feature set, and the easy-to-recognize low-dimensional main feature vector is extracted from the high-dimensional fault feature set; finally, the low-dimensional feature set is imported into the random forest as the input of pattern recognition.
[0121] Further, 6...
Embodiment 2
[0212] Embodiment 2: In this example, the method as shown in Embodiment 1 is adopted to carry out the fault diagnosis of the bearing, and the specific implementation steps are as follows:
[0213] (1) This experiment uses the bearing fault data set collected by the Bearing Data Center of Case Western Reserve University in the United States. The data set is the fan end bearing data at a sampling frequency of 12K. The fault data of 4 different states of normal, inner ring fault, rolling element fault and outer ring fault (6 o'clock direction) are collected respectively. In addition to the normal data, each state has 3 A total of 10 fault types are used as the data source for this experiment. Each class of data is divided into 115 classification samples, and there are 1150 samples in total for 10 classes. The training set size is 700, that is, 70 for each class, and the test set is 450, 45 for each class. The classification is shown in Table 1, where RF, IF, and OF are faults o...
PUM
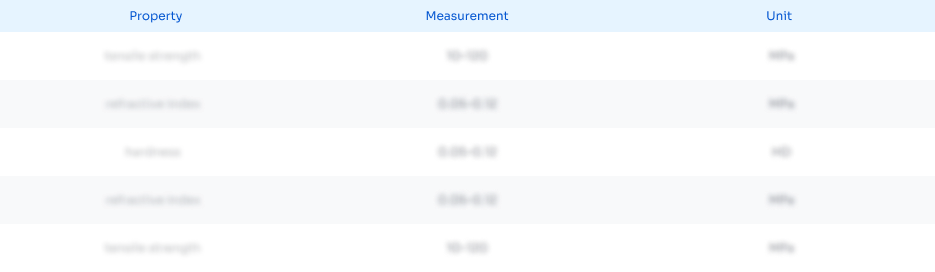
Abstract
Description
Claims
Application Information
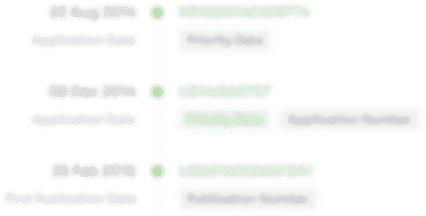
- R&D
- Intellectual Property
- Life Sciences
- Materials
- Tech Scout
- Unparalleled Data Quality
- Higher Quality Content
- 60% Fewer Hallucinations
Browse by: Latest US Patents, China's latest patents, Technical Efficacy Thesaurus, Application Domain, Technology Topic, Popular Technical Reports.
© 2025 PatSnap. All rights reserved.Legal|Privacy policy|Modern Slavery Act Transparency Statement|Sitemap|About US| Contact US: help@patsnap.com