A rolling bearing fault diagnosis method based on improved pso algorithm and optimized fsvm
A rolling bearing and fault diagnosis technology, which is applied in mechanical bearing testing, design optimization/simulation, calculation, etc., can solve the problems of reduced diagnostic accuracy and classification failure to achieve group optimization, so as to improve speed and accuracy, and make the fault diagnosis model reasonable Effective, clear and precise results
- Summary
- Abstract
- Description
- Claims
- Application Information
AI Technical Summary
Problems solved by technology
Method used
Image
Examples
Embodiment 1
[0034] Embodiment 1: as figure 1As shown, a rolling bearing fault diagnosis method based on the improved PSO algorithm to optimize the FSVM, firstly use the wavelet decomposition method to extract the feature vector of the rolling bearing fault data; then use the PSO algorithm to optimize the penalty coefficient and kernel function parameters of the FSVM, Build an optimized FSVM model; then input the extracted feature vectors into the optimized FSVM for training to obtain a rolling bearing fault diagnosis model, and finally use the rolling bearing fault diagnosis model for rolling bearing fault diagnosis model for fault diagnosis. In order to balance the global optimization advantages and local optimization advantages of the particle swarm optimization algorithm model to a greater extent, the present invention will add inertia factors in the process of algorithm optimization, and incorporate the IPSO-FSVM classification model with dynamically updated inertia weights to greatly ...
Embodiment 2
[0054] Embodiment 2: In this embodiment, the method as shown in Embodiment 1 is adopted to carry out the fault diagnosis of the bearing, and the specific implementation steps are as follows:
[0055] According to the wavelet three-layer decomposition principle, the energy characteristics of the bearing are extracted, and some experimental data are shown in Table 1. Four states of bearings (normal, inner ring fault, outer ring fault and rolling element fault) are selected in the experiment, and 30 sets of samples are selected for each type of fault as training samples, so a total of 120 feature vectors need to be selected, and the selected 120 sets of feature vectors The vectors are entered into the data samples in preparation for training. Then select 20 eigenvectors for each kind of fault, a total of 80 are stored in the data sample as the test sample set.
[0056] Table 1. Partial samples of bearing fault energy features extracted by wavelet
[0057]
PUM
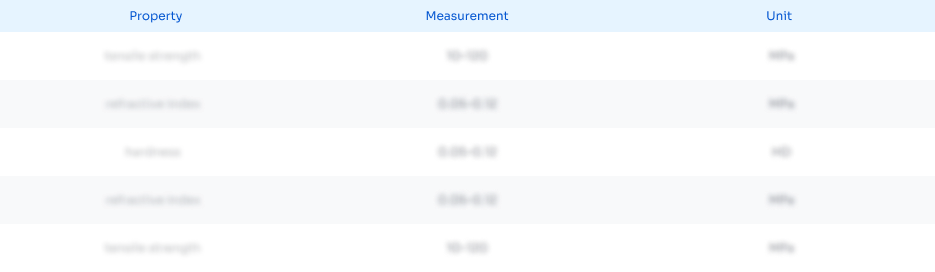
Abstract
Description
Claims
Application Information
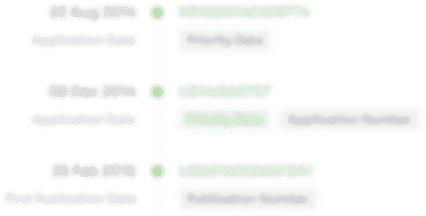
- R&D Engineer
- R&D Manager
- IP Professional
- Industry Leading Data Capabilities
- Powerful AI technology
- Patent DNA Extraction
Browse by: Latest US Patents, China's latest patents, Technical Efficacy Thesaurus, Application Domain, Technology Topic, Popular Technical Reports.
© 2024 PatSnap. All rights reserved.Legal|Privacy policy|Modern Slavery Act Transparency Statement|Sitemap|About US| Contact US: help@patsnap.com