Water surface and underwater target classification method based on interference fringes and deep learning
A technology of interference fringes and deep learning, applied in the fields of marine engineering and underwater acoustic engineering
- Summary
- Abstract
- Description
- Claims
- Application Information
AI Technical Summary
Problems solved by technology
Method used
Image
Examples
Embodiment Construction
[0044] Now in conjunction with embodiment, accompanying drawing, the present invention will be further described:
[0045] The invention proposes to use the Gaussian ray acoustic model BELLHOP and the known SSP simulation to obtain thousands of sound field interference fringe images with different sound source depths, including complete images without interference, fuzzy images and images with random depth errors. Then the blurred image and the image with random depth error are used as the input training set, and the complete image without interference is used as the output training set to train DBN; all the stripe images are used as the input training set, and the category of the image is used as the output training set to train CNN, DBN and CNN needs to be trained thousands of times until it converges. After the training of DBN and CNN, DBN is used as the front-end processing module of CNN, and beamforming is added as a preprocessing module. Finally, a judgment module is add...
PUM
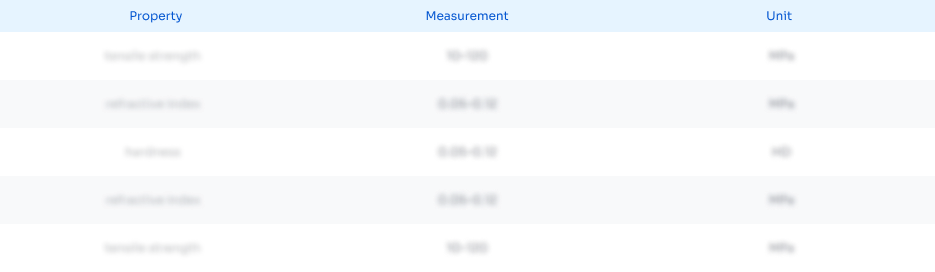
Abstract
Description
Claims
Application Information
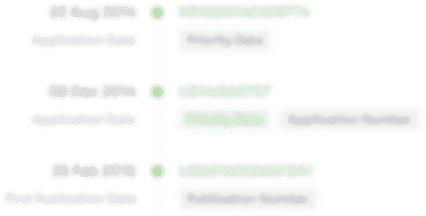
- R&D
- Intellectual Property
- Life Sciences
- Materials
- Tech Scout
- Unparalleled Data Quality
- Higher Quality Content
- 60% Fewer Hallucinations
Browse by: Latest US Patents, China's latest patents, Technical Efficacy Thesaurus, Application Domain, Technology Topic, Popular Technical Reports.
© 2025 PatSnap. All rights reserved.Legal|Privacy policy|Modern Slavery Act Transparency Statement|Sitemap|About US| Contact US: help@patsnap.com