Multi-model fusion method
A fusion method, multi-model technology, applied in character and pattern recognition, instruments, computer parts, etc., can solve the problems of low generalization ability, complex iteration, training not helpful, etc. Reduce the effect of iterative complexity and strong generalization ability
- Summary
- Abstract
- Description
- Claims
- Application Information
AI Technical Summary
Problems solved by technology
Method used
Image
Examples
Embodiment
[0049] The XGBoost+LR fusion model processing flow includes two processes, each of which includes four processes including data preparation, feature engineering, model training, and result output, such as figure 1 As shown, for example: the data preparation (that is, data processing) step is mainly to clean and reshape the data set; the feature engineering is derived from the user's multi-dimensional features, and is selected through feature importance, and the top 100 features are respectively Pass in two XGBoost models, and after traversing 400 trees, record the number of leaf nodes of the training set samples in each tree (referring to the tuned XGBoost model, the number of training set samples in the leaf nodes of each tree), and pass One-hot encoding conversion to obtain all the LR features corresponding to the sample, then train and tune the LR model, and output the model result, which is the improved Gaussian transformation of the result. Specific steps are as follows:...
PUM
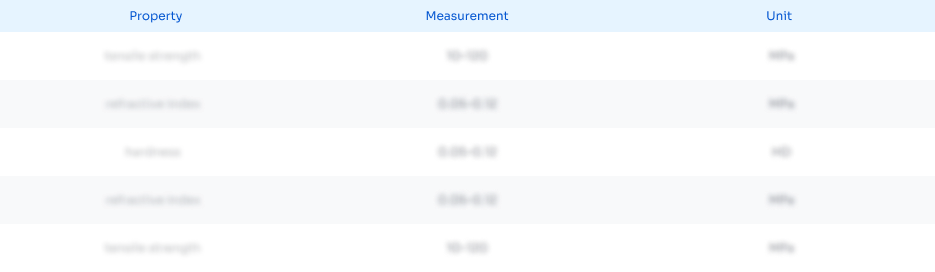
Abstract
Description
Claims
Application Information
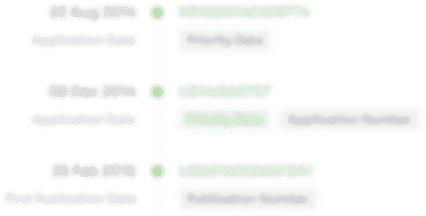
- R&D Engineer
- R&D Manager
- IP Professional
- Industry Leading Data Capabilities
- Powerful AI technology
- Patent DNA Extraction
Browse by: Latest US Patents, China's latest patents, Technical Efficacy Thesaurus, Application Domain, Technology Topic.
© 2024 PatSnap. All rights reserved.Legal|Privacy policy|Modern Slavery Act Transparency Statement|Sitemap