Bank electronic channel abnormal transaction determination method based on semi-supervised learning
A semi-supervised learning, abnormal transaction technology, applied in the field of abnormal transaction determination in bank electronic channels, can solve problems such as poor accuracy, high difficulty, and low efficiency, and achieve the effect of reducing difficulty, improving work efficiency, and improving accuracy.
- Summary
- Abstract
- Description
- Claims
- Application Information
AI Technical Summary
Problems solved by technology
Method used
Image
Examples
specific Embodiment approach 1
[0033] Specific implementation mode one: the following combination figure 1 This embodiment is described. In this embodiment, a semi-supervised learning-based method for determining abnormal transactions in bank electronic channels includes the following steps:
[0034] Step 1: Input a labeled sample set L and an unlabeled sample set U, perform SMUC clustering on L and U, and select the first r unlabeled samples with a high degree of membership to construct a sample set R 1 , if there are less than r unlabeled samples with high membership degree, use these unlabeled samples directly to construct R 1 , where r is the number of unlabeled samples selected after SMUC clustering, and k is the nearest neighbor parameter;
[0035] Step 2: Use L to train the classifier h on two different views 1 and h 2 , use the trained classifier to R 1 Classification;
[0036] Step 3: Determine h 1 、h 2 to R 1 Whether the classification of each sample in is consistent, if the record is cons...
specific Embodiment approach 2
[0080] Embodiment 2: This embodiment is a further description of Embodiment 1. The difference between this embodiment and Embodiment 1 is that the specific steps of SMUC clustering in Step 1 are:
[0081] Step 11: Initialize the dataset;
[0082] Step 1 and 2: Initialize the membership array;
[0083] Step 13: update the cluster center according to the membership array;
[0084] Step 14: update the membership degree array according to the cluster center;
[0085] Step 15: Determine whether the end condition is met, that is, the center of mass does not change any more, if not, repeat steps 12 to 14.
specific Embodiment approach 3
[0086] Specific embodiment three: this embodiment is a further description of specific embodiment one, and the difference between this embodiment and specific embodiment one is the specific steps of the Baum-Welch algorithm in the described step six:
[0087] Step 61: Randomly set the initial model M 0 ;
[0088] Step 62: Based on M 0 And the sequence of observations O, train a new model M *;
[0089] Step 63: If the condition is satisfied||M * -M 0 ||<ε, that is, the training has reached the expected effect, and it is over. If it is not satisfied, go to step 64;
[0090] Step 64: Let M 0 = M * , continue to work in step 62.
PUM
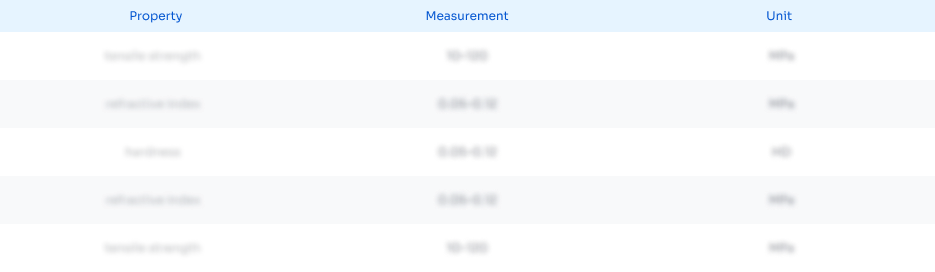
Abstract
Description
Claims
Application Information
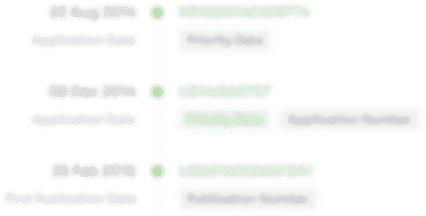
- R&D Engineer
- R&D Manager
- IP Professional
- Industry Leading Data Capabilities
- Powerful AI technology
- Patent DNA Extraction
Browse by: Latest US Patents, China's latest patents, Technical Efficacy Thesaurus, Application Domain, Technology Topic, Popular Technical Reports.
© 2024 PatSnap. All rights reserved.Legal|Privacy policy|Modern Slavery Act Transparency Statement|Sitemap|About US| Contact US: help@patsnap.com