Semantic segmentation method and system based on edge dense reconstruction for streetscape understanding
A semantic segmentation and edge technology, applied in the field of computer vision, can solve problems such as failure to solve the problem of object boundaries, failure to effectively reuse features, and blurring of objects in a targeted manner.
- Summary
- Abstract
- Description
- Claims
- Application Information
AI Technical Summary
Problems solved by technology
Method used
Image
Examples
Embodiment Construction
[0045] The present invention will be further described in detail below in conjunction with the drawings and specific embodiments.
[0046] The present invention provides a semantic segmentation method based on dense edge reconstruction for street scene understanding, such as figure 1 As shown, including the following steps:
[0047] Step A: Preprocess the input images of the training set. First, subtract the image average from the images to standardize them, and then randomly cut the images with uniform sizes to obtain preprocessed images of the same size.
[0048] Step B: Use a general convolutional network to extract general features F backbone , And then based on the general feature F backbone Obtain the three-level context space pyramid fusion feature F tspp , Used to capture multi-scale context information, and then use the cascade of the two parts described in step B as a coding network to extract coding features F encoder ; It includes the following steps:
[0049] Step B1: U...
PUM
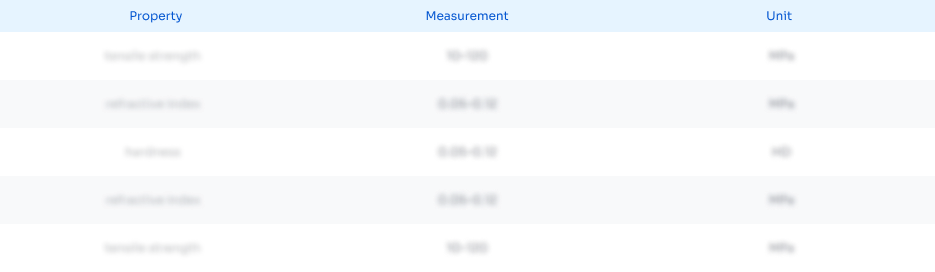
Abstract
Description
Claims
Application Information
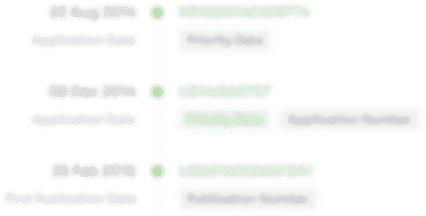
- Generate Ideas
- Intellectual Property
- Life Sciences
- Materials
- Tech Scout
- Unparalleled Data Quality
- Higher Quality Content
- 60% Fewer Hallucinations
Browse by: Latest US Patents, China's latest patents, Technical Efficacy Thesaurus, Application Domain, Technology Topic, Popular Technical Reports.
© 2025 PatSnap. All rights reserved.Legal|Privacy policy|Modern Slavery Act Transparency Statement|Sitemap|About US| Contact US: help@patsnap.com