Real-time image semantic segmentation method based on lightweight full convolutional neural network
A convolutional neural network and full convolutional network technology, applied in the field of computer software, can solve problems such as difficult mobile platform operation, poor classification accuracy, and method defects, and achieve the effect of reducing memory usage and calculation data volume
- Summary
- Abstract
- Description
- Claims
- Application Information
AI Technical Summary
Problems solved by technology
Method used
Image
Examples
Embodiment Construction
[0026] The invention proposes a real-time image semantic segmentation method based on a lightweight full convolutional neural network. Three network module structures adapted to semantic segmentation tasks are proposed. After training and testing on two data sets of CamVid and Cityscapes, not only the model scale is controlled, but also high-precision semantic segmentation results are obtained under real-time prediction speed.
[0027] The implementation steps of the present invention are as follows:
[0028] 1) Use the design elements of lightweight neural network to build a fully convolutional neural network, lightweight network models such as MobileNetV2, ShuffleNetV2, etc. The network designed by the present invention includes three stages: feature extension stage, feature processing stage, and comprehensive prediction stage, such as figure 1 Shown.
[0029] The feature extension stage is divided into two parallel extraction features. One way uses two extraction processing modu...
PUM
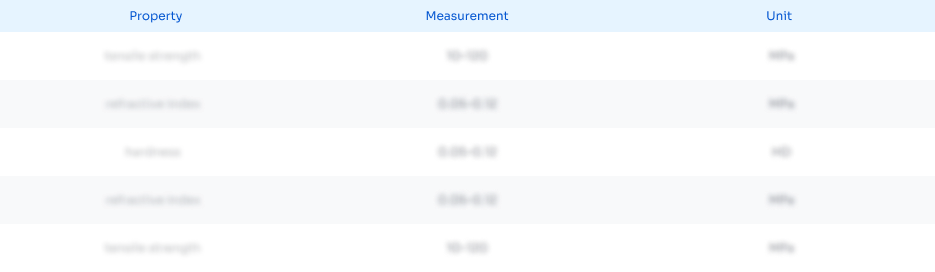
Abstract
Description
Claims
Application Information
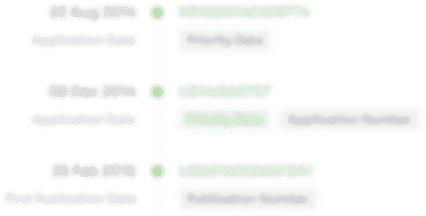
- R&D Engineer
- R&D Manager
- IP Professional
- Industry Leading Data Capabilities
- Powerful AI technology
- Patent DNA Extraction
Browse by: Latest US Patents, China's latest patents, Technical Efficacy Thesaurus, Application Domain, Technology Topic, Popular Technical Reports.
© 2024 PatSnap. All rights reserved.Legal|Privacy policy|Modern Slavery Act Transparency Statement|Sitemap|About US| Contact US: help@patsnap.com