Medical insurance data analysis method based on big data
A data analysis and big data technology, applied in data processing applications, healthcare informatics, patient care, etc., can solve problems such as inability to classify big data and establish effective medical insurance prediction models, so as to improve data accuracy and guarantee Accuracy and timeliness, the effect of good data integration
- Summary
- Abstract
- Description
- Claims
- Application Information
AI Technical Summary
Problems solved by technology
Method used
Image
Examples
Embodiment 1
[0052] A method for analyzing medical insurance data based on big data, comprising the following steps;
[0053] S1. Organize medical insurance data in a certain area, including validity check, error data elimination and modification;
[0054] S2. Preliminary statistics, forming a preliminary understanding of the fluctuation law. Classify the electricity consumption data of different industries and regions, analyze the trend of the data curve according to the characteristics of different data, and combine the preliminary autocorrelation analysis to study the internal and external factors that lead to the irregular changes of major diseases and staged infectious diseases. When sorting out different data studies, integrate them according to monthly, quarterly and annual time spans;
[0055] S3. Construction of medical insurance data forecasting model, searching for forecasting models with high forecasting accuracy and goodness of fit by categories and scales, monthly, quarterly...
Embodiment 2
[0089] Embodiment 2: Based on Embodiment 1 but different;
[0090] Monthly forecast error of medical insurance in a certain area
[0091]
[0092] Quarterly forecast error of medical insurance in a certain area
[0093]
[0094] Annual forecast error of medical insurance in a certain area
[0095]
[0096] Through the representative models in the model library, the medical insurance data in a certain area are fitted and predicted. The results show that choosing the ARIMA model for monthly and quarterly data can get better prediction results. For annual data, choosing the gray system model can get better prediction results. prediction results.
Embodiment 3
[0097] Embodiment 3: based on embodiment 1 and 2 but different;
[0098] Through the analysis of the medical insurance situation of various population groups in this area, a population classification method based on monthly medical insurance sequence clustering is proposed. The analysis results of various influencing factors show that the classification results obtained by this classification method are stable and reliable. Based on the classification results obtained, this study analyzes the proportion of medical insurance among various groups of people and the impact of medical insurance fluctuations, and selects 10 specific groups in three categories as the key medical insurance groups. These 10 groups have the largest proportion of medical insurance among all groups, and at the same time have the greatest impact on the fluctuation of the overall medical insurance. Based on the classification of medical insurance groups, a prediction model of medical insurance demand by po...
PUM
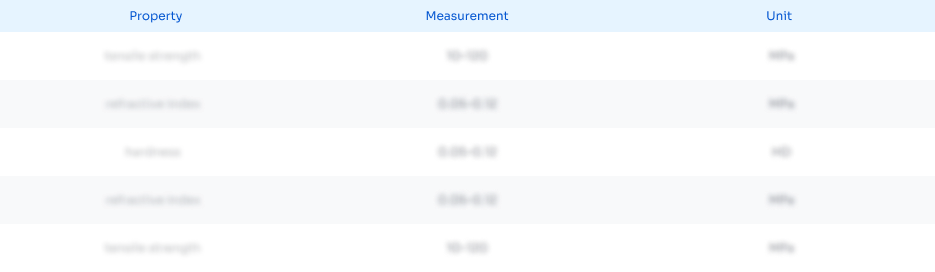
Abstract
Description
Claims
Application Information
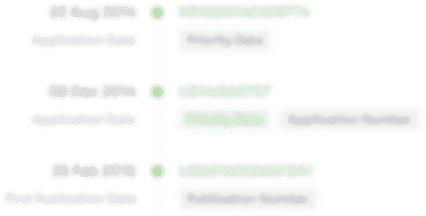
- R&D Engineer
- R&D Manager
- IP Professional
- Industry Leading Data Capabilities
- Powerful AI technology
- Patent DNA Extraction
Browse by: Latest US Patents, China's latest patents, Technical Efficacy Thesaurus, Application Domain, Technology Topic, Popular Technical Reports.
© 2024 PatSnap. All rights reserved.Legal|Privacy policy|Modern Slavery Act Transparency Statement|Sitemap|About US| Contact US: help@patsnap.com