Training method and segmentation method and apparatus for a segmentation learning network of a 3D image and medium
A technology for learning networks and training methods, applied in the field of non-transitory computer-readable media, which can solve problems such as overfitting of learning networks, scarcity of training samples, imbalance of foreground and background, etc., achieve high training success rate and avoid overfitting Problem, effect of fast and accurate segmentation
- Summary
- Abstract
- Description
- Claims
- Application Information
AI Technical Summary
Problems solved by technology
Method used
Image
Examples
Embodiment Construction
[0025] image 3 A flowchart of a training method 300 of a segmentation learning network for a 3D image according to an embodiment of the present disclosure is shown. The segmentation learning network and its training method are especially suitable for 3D images whose object of interest occupies less than a predetermined threshold (the foreground and background are out of balance to some extent). In some embodiments, the predetermined threshold is in the range of 0.0001% to 30%. For example, 3D MRI images of the brain containing nasopharyngeal carcinoma or nasopharyngeal staging tumors, 3D volumetric CT images of the abdomen containing small lung nodules, and whole body 3D containing early lesions of small and irregularly shaped unknown parts Volume CT images and so on.
[0026] Such as image 3 As shown, the method 300 starts at step 301, which constructs the segmentation learning network based on the sequential joint of a plurality of dense blocks. The basic units in the dense bl...
PUM
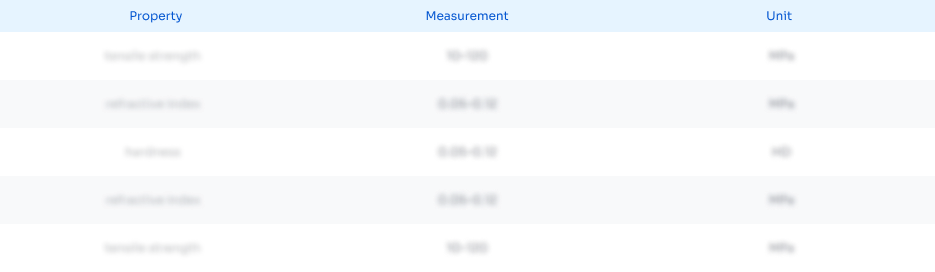
Abstract
Description
Claims
Application Information
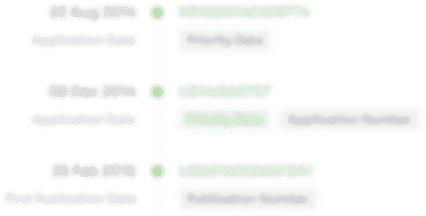
- R&D Engineer
- R&D Manager
- IP Professional
- Industry Leading Data Capabilities
- Powerful AI technology
- Patent DNA Extraction
Browse by: Latest US Patents, China's latest patents, Technical Efficacy Thesaurus, Application Domain, Technology Topic, Popular Technical Reports.
© 2024 PatSnap. All rights reserved.Legal|Privacy policy|Modern Slavery Act Transparency Statement|Sitemap|About US| Contact US: help@patsnap.com