A flood forecasting method based on depth learning model and BP neural network correction
A BP neural network and deep learning technology, which is applied in the field of flood prediction based on deep learning models and BP neural network corrections, can solve problems such as complex models, poor model adaptability, and difficult calibration of model parameters, so as to improve the accuracy rate , easy to set up, and improve the effect of peak time and peak forecast accuracy
- Summary
- Abstract
- Description
- Claims
- Application Information
AI Technical Summary
Problems solved by technology
Method used
Image
Examples
Embodiment
[0079] In order to verify the effect of the present invention, the Tunxi River Basin in Anhui Province was selected, and a model was built to predict the flood process in the watershed. The catchment area of the watershed is 2696.76 square kilometers. It is located in a subtropical monsoon climate, with a suitable climate, an annual average temperature of 17°C, and abundant rainfall. The data of 33 floods that occurred from 1982 to 2002 were selected as the research data of the experiment. All data have been compiled and processed through hydrological data, and there is no missing data.
[0080] The following is a comparative experiment from the two aspects of the forecast period and real-time correction results, to analyze the experimental results and test the usability of the model.
[0081] 1) Starting from the foreseeable period of the experimental model. Taking the forecast period as the starting point to design a comparative experiment, carry out traffic forecasting, ...
PUM
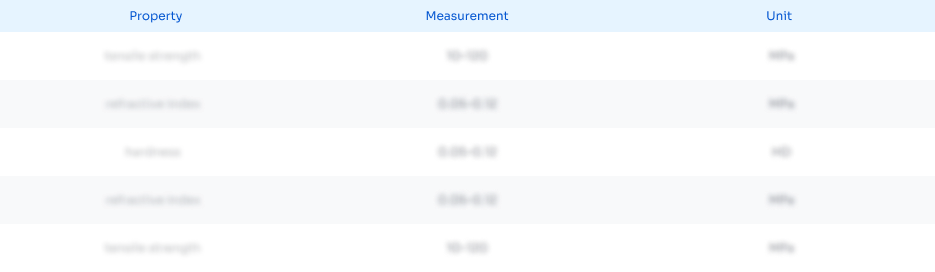
Abstract
Description
Claims
Application Information
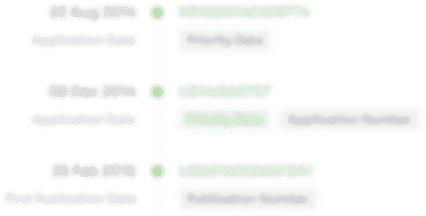
- R&D Engineer
- R&D Manager
- IP Professional
- Industry Leading Data Capabilities
- Powerful AI technology
- Patent DNA Extraction
Browse by: Latest US Patents, China's latest patents, Technical Efficacy Thesaurus, Application Domain, Technology Topic, Popular Technical Reports.
© 2024 PatSnap. All rights reserved.Legal|Privacy policy|Modern Slavery Act Transparency Statement|Sitemap|About US| Contact US: help@patsnap.com