Whole vehicle load prediction method based on LSTM neural network
A technology of neural network and prediction method, which is applied in the direction of neural learning method, biological neural network model, prediction, etc., which can solve the problems of easy aging of sensors, slow convergence speed, increased maintenance cost, etc., so as to save later maintenance cost and improve prediction accuracy , The effect of saving manufacturing cost
- Summary
- Abstract
- Description
- Claims
- Application Information
AI Technical Summary
Problems solved by technology
Method used
Image
Examples
Embodiment Construction
[0033] The present invention will be further described below in conjunction with drawings and embodiments.
[0034] The present invention provides a kind of vehicle load forecasting method based on LSTM neural network, comprising the following steps:
[0035] (1) Collect vehicle data through the vehicle terminal (T-BOX), including time, vehicle speed, engine speed, clutch switch, accelerator pedal opening and brake switch, and torque or torque percentage;
[0036] (2) Integrate, clean and standardize the vehicle data to obtain training data;
[0037] This step is mainly to prepare the data. From the longitudinal dynamics formula:
[0038]
[0039] Among them, M represents the total mass of the car, v represents the driving speed of the car (data can be collected), acceleration T e Indicates the torque of the engine acting on the flywheel (data can be collected), Indicates the overall gear ratio, where r w Indicates the radius of the wheel (static value of the model)...
PUM
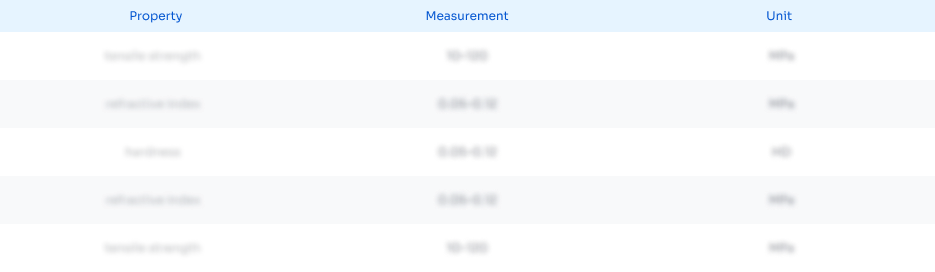
Abstract
Description
Claims
Application Information
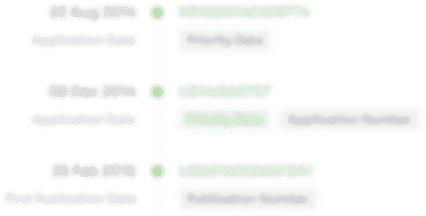
- R&D
- Intellectual Property
- Life Sciences
- Materials
- Tech Scout
- Unparalleled Data Quality
- Higher Quality Content
- 60% Fewer Hallucinations
Browse by: Latest US Patents, China's latest patents, Technical Efficacy Thesaurus, Application Domain, Technology Topic, Popular Technical Reports.
© 2025 PatSnap. All rights reserved.Legal|Privacy policy|Modern Slavery Act Transparency Statement|Sitemap|About US| Contact US: help@patsnap.com