Model design method based on depth nearest class mean value and incremental smell classification method
A mean value model and depth technology, applied in neural learning methods, biological neural network models, computing, etc., can solve problems such as being unsuitable for highly nonlinear classification tasks, and achieve the effect of wide application range and small calculation amount.
- Summary
- Abstract
- Description
- Claims
- Application Information
AI Technical Summary
Problems solved by technology
Method used
Image
Examples
Embodiment 1
[0063] Such as image 3 As shown, Embodiment 1 of the present invention provides a method for designing a deep nearest class mean model, and the method includes the following steps:
[0064] S101. Design a deep neural network model; wherein, the deep neural network model includes: an input layer, at least one hidden layer, a fully connected layer, and a Softmax functional layer;
[0065] S102. Replace the fully connected layer in the deep neural network model with an NCM layer to obtain a deep nearest class mean model.
[0066] Preferably, after the step of replacing the fully connected layer in the deep neural network model with an NCM layer to obtain the depth nearest class mean model, the method also includes the following model training steps:
[0067] Select training data and test data of the nearest class mean model of depth; Wherein, the training data includes initial training phase data and update training phase data, and the test data includes initial testing phase d...
Embodiment 2
[0078] Such as Figure 4 As shown, Embodiment 2 of the present invention provides an incremental odor classification method based on the depth nearest class mean model, which includes all the contents of the design method of a depth nearest class mean model described in Embodiment 1, and also includes The following steps:
[0079] S201. Collect odor data;
[0080] S202. Sampling the collected odor data through the sensor array to obtain sensor array signals;
[0081] S203. Input the odor data into the pre-trained deep closest mean class model, and perform feature classification by the Softmax function layer in the deep closest mean class model.
[0082] The embodiment of the present invention provides an incremental odor classification method based on the deep nearest class mean model, which combines the advantages of the deep neural network and the nearest class mean classifier, and eliminates their respective limitations, and can seamlessly accommodate new odor categories...
PUM
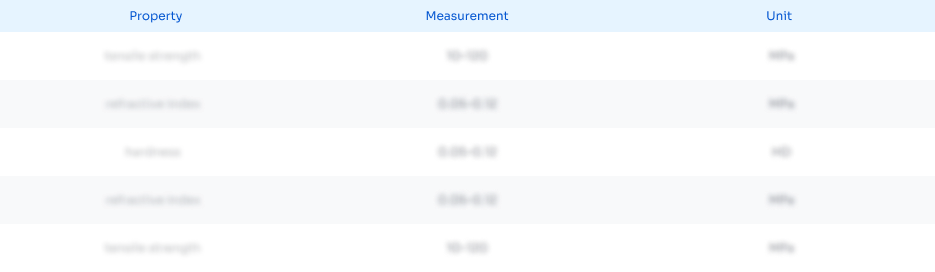
Abstract
Description
Claims
Application Information
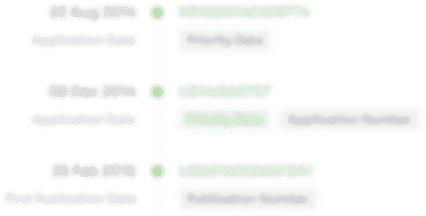
- R&D Engineer
- R&D Manager
- IP Professional
- Industry Leading Data Capabilities
- Powerful AI technology
- Patent DNA Extraction
Browse by: Latest US Patents, China's latest patents, Technical Efficacy Thesaurus, Application Domain, Technology Topic, Popular Technical Reports.
© 2024 PatSnap. All rights reserved.Legal|Privacy policy|Modern Slavery Act Transparency Statement|Sitemap|About US| Contact US: help@patsnap.com