Multi-target unmarked attitude estimation method based on deep convolutional neural network
A pose estimation and neural network technology, applied in the field of computer vision, can solve problems such as difficulty in the use of personnel, camera distortion, uneven lighting, etc., and achieve the effects of less loss of prediction accuracy, improved accuracy, and good robustness
- Summary
- Abstract
- Description
- Claims
- Application Information
AI Technical Summary
Problems solved by technology
Method used
Image
Examples
Embodiment
[0054] The multi-target unmarked attitude estimation method based on deep convolutional neural network of the present invention comprises the following contents:
[0055] 1. Acquire image sequences containing target behaviors from videos. Specifically:
[0056] Using the clustering method of visual appearance (K-means), collect image sequences of the behavior of the target of interest under different brightness conditions and background conditions, and ensure that the training data set contains a sufficient number of image sequences (100-200). The image sequence collected in this embodiment is as follows figure 2 shown.
[0057] 2. For each image in the collected image sequence, manually mark the position and category of each target feature part in the same order, and construct a training data set and a test data set according to the marked image sequence. Specifically:
[0058] 70% of the image sequences are randomly selected as the training image set, and the remaining ...
PUM
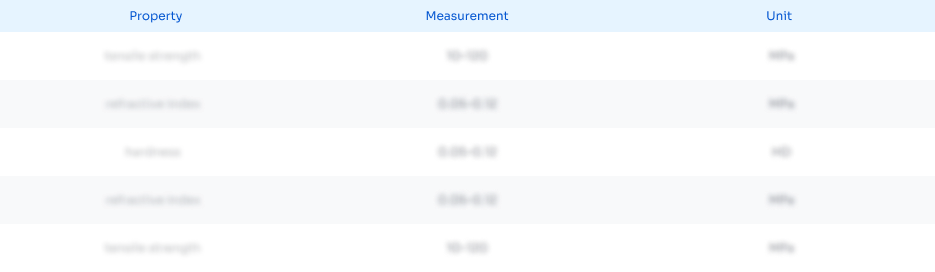
Abstract
Description
Claims
Application Information
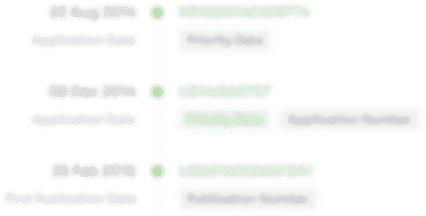
- R&D Engineer
- R&D Manager
- IP Professional
- Industry Leading Data Capabilities
- Powerful AI technology
- Patent DNA Extraction
Browse by: Latest US Patents, China's latest patents, Technical Efficacy Thesaurus, Application Domain, Technology Topic.
© 2024 PatSnap. All rights reserved.Legal|Privacy policy|Modern Slavery Act Transparency Statement|Sitemap