Generative adversarial migration learning method based on sketch annotation information
A technology of labeling information and transfer learning, applied in the field of cross-domain image classification, which can solve the problem of inability to judge the invariant features of the feature domain.
- Summary
- Abstract
- Description
- Claims
- Application Information
AI Technical Summary
Problems solved by technology
Method used
Image
Examples
Embodiment Construction
[0073]The present invention provides a generation confrontational transfer learning method based on sketch annotation information, which acquires an initial sketch map and constructs a paired data set in the form of "source domain image-source domain image edge annotation map"; constructs an edge based on sketch annotation information Segment and train the deep network; select target domain samples based on matrix norm; construct and train a generation-adversarial transfer learning network based on sketch annotation information, which includes a deep generator network, a deep discriminator network, and an edge segmentation depth based on sketch annotation information Network and deep classifier network; input the target domain image, and obtain the classification result of the target domain image; the present invention utilizes the similarity of the source domain data and the target domain data structure, and through structural constraints, generates samples that conform to the ...
PUM
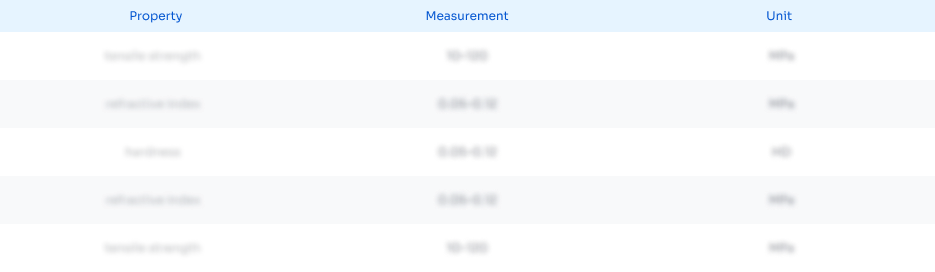
Abstract
Description
Claims
Application Information
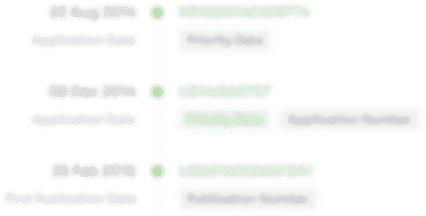
- R&D Engineer
- R&D Manager
- IP Professional
- Industry Leading Data Capabilities
- Powerful AI technology
- Patent DNA Extraction
Browse by: Latest US Patents, China's latest patents, Technical Efficacy Thesaurus, Application Domain, Technology Topic, Popular Technical Reports.
© 2024 PatSnap. All rights reserved.Legal|Privacy policy|Modern Slavery Act Transparency Statement|Sitemap|About US| Contact US: help@patsnap.com