Semantic visual dictionary optimization method based on Pearson correlation coefficients
A Pearson correlation and visual dictionary technology, applied in the field of semantic visual dictionary optimization based on Pearson correlation coefficient, can solve problems such as increasing the time cost of computer classification, increasing the time complexity of image classification, and affecting the efficiency of image noise recognition. Effects of semantic gap and redundancy, shortened computation time, and small dimensions
- Summary
- Abstract
- Description
- Claims
- Application Information
AI Technical Summary
Problems solved by technology
Method used
Image
Examples
Embodiment Construction
[0025] The present invention will be further described below in conjunction with the accompanying drawings.
[0026] 1. Suppose the training image set is D=[d 1 , d 2 ,...,d i ,...,d N ], where d i represents the i-th image.
[0027] 2. Use the SURF algorithm to extract the image dynamic feature set R={r 1 , r 2 ,...,r i ,...,r N-1 , r N}, where r i is a dynamic feature of the image, and N is the number of features in the dynamic feature set R;
[0028] 3. Use the precise European locality-sensitive hash clustering algorithm to cluster the dynamic features of the acquired images to generate a hash table T g ={b 1 , b 2 ,...,b k ,...,b Z}, where b k Indicates the kth bucket in the hash table, Z indicates the total number of buckets in the hash table, and the hash table T g Complete a specific division of image dynamic features, hash table T g ={b 1 , b 2 ,...,b k ,...,b Z} is the original visual dictionary. Specific steps are as follows:
[0029] a. From...
PUM
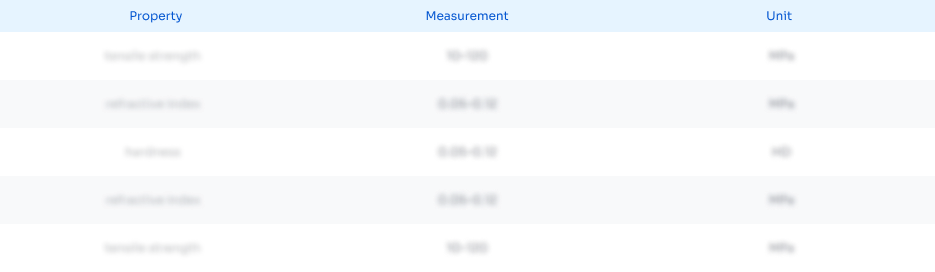
Abstract
Description
Claims
Application Information
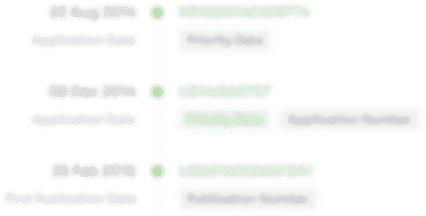
- R&D Engineer
- R&D Manager
- IP Professional
- Industry Leading Data Capabilities
- Powerful AI technology
- Patent DNA Extraction
Browse by: Latest US Patents, China's latest patents, Technical Efficacy Thesaurus, Application Domain, Technology Topic.
© 2024 PatSnap. All rights reserved.Legal|Privacy policy|Modern Slavery Act Transparency Statement|Sitemap