Hyperspectral anomaly detection method and system based on manifold constraint self-coding network
A self-encoding network and anomaly detection technology, applied in biological neural network models, optical device exploration, image data processing, etc., can solve the problems of no consideration, small proportion of abnormal targets, and no consideration of local characteristics of hyperspectral data, etc. The effect of improving detection rate and improving detection ability
- Summary
- Abstract
- Description
- Claims
- Application Information
AI Technical Summary
Problems solved by technology
Method used
Image
Examples
Embodiment Construction
[0039] The present invention will be further described below in conjunction with the accompanying drawings and specific embodiments.
[0040] The steps that the present invention realizes are as follows:
[0041] Step 1, input the hyperspectral image X to be detected, and learn the low-dimensional embedding manifold Y of the hyperspectral image by using the manifold learning method local linear embedding;
[0042] Step 2, input the hyperspectral image X to be detected, pre-train the manifold constrained autoencoder network layer by layer, and its loss function is
[0043]
[0044] where x i is the i-th sample, is the i-th reconstructed sample. W (l) is the weight coefficient between layer l and layer l+1. λ is the weight decay parameter. ρ is a sparse parameter, which is a positive number very close to 0. s is the number of neurons in the hidden layer. is the average response value of the jth neuron. is the KL divergence.
[0045] Step 3, use the parameters obt...
PUM
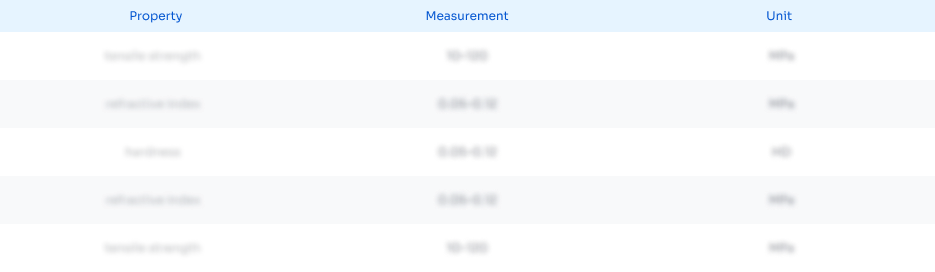
Abstract
Description
Claims
Application Information
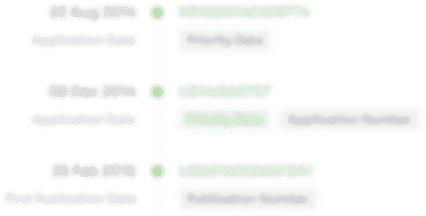
- R&D Engineer
- R&D Manager
- IP Professional
- Industry Leading Data Capabilities
- Powerful AI technology
- Patent DNA Extraction
Browse by: Latest US Patents, China's latest patents, Technical Efficacy Thesaurus, Application Domain, Technology Topic, Popular Technical Reports.
© 2024 PatSnap. All rights reserved.Legal|Privacy policy|Modern Slavery Act Transparency Statement|Sitemap|About US| Contact US: help@patsnap.com