Cross-modal deep hash retrieval method based on self-supervision
A modal and hashing technology, applied in the field of cross-modal deep hash retrieval, can solve the problems of insufficient training, disappearance of neural network gradients, and insufficient use of label data, and achieve the effect of excellent retrieval performance.
- Summary
- Abstract
- Description
- Claims
- Application Information
AI Technical Summary
Problems solved by technology
Method used
Image
Examples
Embodiment Construction
[0017] The present invention proposes a cross-modal deep Hiha retrieval method based on self-supervision. Specific embodiments of the present invention will be described in detail below in conjunction with the accompanying drawings.
[0018] This cross-modal deep hash retrieval method constructs an independent category label processing network in a self-supervised manner to learn the semantic features of the label data, model the semantic features of data between different modalities, and supervise the image and text networks at the same time. The extracted features make their semantic feature distribution tend to be consistent, so that the obtained hash code can better retain semantic information. At the same time, in view of the data imbalance problem in the training data set, a loss function of adaptive weight is proposed, and the weight is automatically adjusted according to the proportion of relevant and irrelevant samples in the samples input to the network each time, so...
PUM
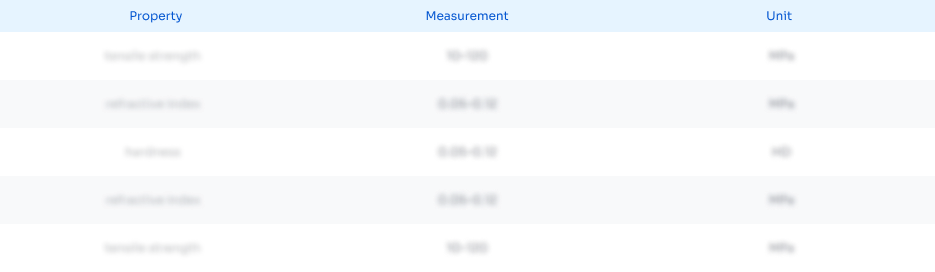
Abstract
Description
Claims
Application Information
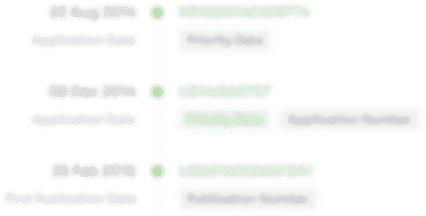
- R&D Engineer
- R&D Manager
- IP Professional
- Industry Leading Data Capabilities
- Powerful AI technology
- Patent DNA Extraction
Browse by: Latest US Patents, China's latest patents, Technical Efficacy Thesaurus, Application Domain, Technology Topic.
© 2024 PatSnap. All rights reserved.Legal|Privacy policy|Modern Slavery Act Transparency Statement|Sitemap