Road surface roughness prediction method based on random forest
A technology of random forest algorithm and prediction method, applied in the field of road surface monitoring, can solve the problems of decreased fault tolerance, low network performance, slow prediction speed, etc., to achieve the effect of improving prediction accuracy, improving prediction accuracy and speeding up
- Summary
- Abstract
- Description
- Claims
- Application Information
AI Technical Summary
Problems solved by technology
Method used
Image
Examples
Embodiment Construction
[0028] A kind of road surface roughness prediction method based on random forest that the present invention provides, specifically comprises the following steps:
[0029] Step 1: Collect asphalt pavement samples. For each asphalt pavement in the asphalt pavement sample, collect the road surface roughness index IRI and the values of 27 pavement parameters to obtain the asphalt pavement sample data.
[0030] Among them, 27 pavement parameters include: mild cracks, moderate cracks, severe cracks, mild massive cracks, severe massive cracks, mild edge cracks, mild longitudinal cracks, moderate longitudinal cracks, Mild non-wheel longitudinal cracks, moderate non-wheel longitudinal cracks, severe non-wheel longitudinal cracks, mild transverse cracks, moderate transverse cracks, severe transverse cracks, light repairs, moderate repairs, heavy repairs, severe potholes, pan Oil, loose, total annual precipitation, annual average temperature, freezing index, annual average daily traffi...
PUM
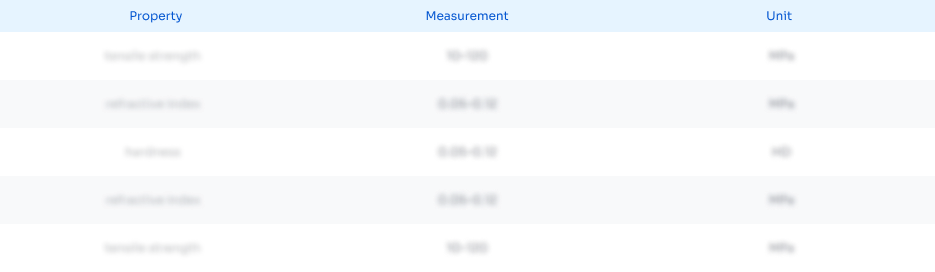
Abstract
Description
Claims
Application Information
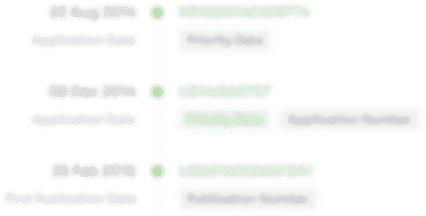
- R&D
- Intellectual Property
- Life Sciences
- Materials
- Tech Scout
- Unparalleled Data Quality
- Higher Quality Content
- 60% Fewer Hallucinations
Browse by: Latest US Patents, China's latest patents, Technical Efficacy Thesaurus, Application Domain, Technology Topic, Popular Technical Reports.
© 2025 PatSnap. All rights reserved.Legal|Privacy policy|Modern Slavery Act Transparency Statement|Sitemap|About US| Contact US: help@patsnap.com