Convolutional neural network quantification method and device, computer and storage medium
A technology of convolutional neural network and quantization method, applied in neural learning methods, biological neural network models, neural architectures, etc., can solve the problems of complex implementation and high computational complexity, and can ensure acceleration effect, reduce storage space, and ensure performance. Effect
- Summary
- Abstract
- Description
- Claims
- Application Information
AI Technical Summary
Problems solved by technology
Method used
Image
Examples
Embodiment Construction
[0045] The present invention will be described in detail below in conjunction with specific embodiments. The following examples will help those skilled in the art to further understand the present invention, but do not limit the present invention in any form. It should be noted that those skilled in the art can make several modifications and improvements without departing from the concept of the present invention. These all belong to the protection scope of the present invention.
[0046] refer to figure 1 As shown, the flowchart of the compression and acceleration method of the convolutional neural network in the embodiment of the present invention can refer to the following steps for details:
[0047] S1, train the full-precision model of the convolutional neural network to be quantized, and calculate the standard deviation of the weight of each layer of the full-precision model and the standard deviation of the response distribution of each layer of the full-precision mod...
PUM
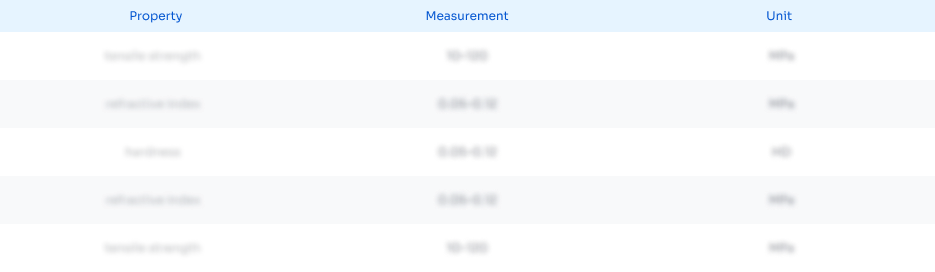
Abstract
Description
Claims
Application Information
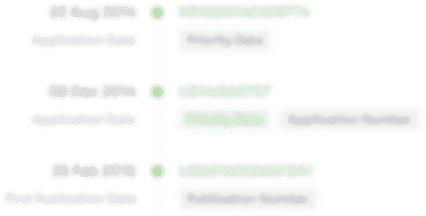
- Generate Ideas
- Intellectual Property
- Life Sciences
- Materials
- Tech Scout
- Unparalleled Data Quality
- Higher Quality Content
- 60% Fewer Hallucinations
Browse by: Latest US Patents, China's latest patents, Technical Efficacy Thesaurus, Application Domain, Technology Topic, Popular Technical Reports.
© 2025 PatSnap. All rights reserved.Legal|Privacy policy|Modern Slavery Act Transparency Statement|Sitemap|About US| Contact US: help@patsnap.com