Methods, apparatus, and systems for estimating causal relationships between observed variables
A causal relationship and variable technology, applied in the field of data mining, can solve problems such as high time complexity, reduced accuracy of causal structure learning, and inability to support complex causal structure learning of observed variable dimensions, and achieve the effect of accelerated solution and accurate causal relationship
- Summary
- Abstract
- Description
- Claims
- Application Information
AI Technical Summary
Problems solved by technology
Method used
Image
Examples
Embodiment Construction
[0026] Hereinafter, various exemplary embodiments of the present disclosure will be described in detail with reference to the accompanying drawings. It should be noted that these drawings and description relate to preferred embodiments as examples only. It should be noted that, from the ensuing description, alternative embodiments of the structures and methods disclosed herein are readily conceivable and may be employed without departing from the disclosed principles of the present disclosure as claimed.
[0027] It should be understood that these exemplary embodiments are given only to enable those skilled in the art to better understand and implement the present disclosure, but not to limit the scope of the present disclosure in any way. In addition, in the drawings, for the purpose of illustration, optional steps, modules, modules, etc. are shown in dashed boxes.
[0028]The terms "including", "comprising" and similar terms used herein should be understood as open-ended te...
PUM
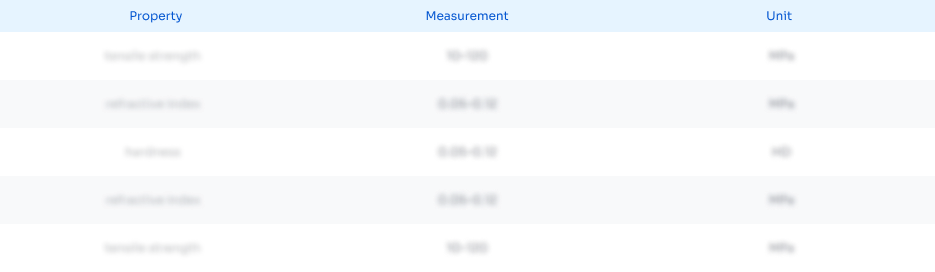
Abstract
Description
Claims
Application Information
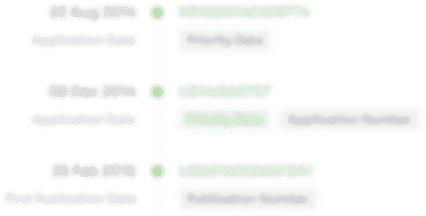
- R&D Engineer
- R&D Manager
- IP Professional
- Industry Leading Data Capabilities
- Powerful AI technology
- Patent DNA Extraction
Browse by: Latest US Patents, China's latest patents, Technical Efficacy Thesaurus, Application Domain, Technology Topic.
© 2024 PatSnap. All rights reserved.Legal|Privacy policy|Modern Slavery Act Transparency Statement|Sitemap