Hierarchical Bagging method for sentiment analysis based on electroencephalogram signals
A technology of EEG signal and emotion analysis, applied in the direction of instruments, character and pattern recognition, computer components, etc., can solve the problems of sampling error, unsuitable algorithm, elimination, etc., to improve accuracy, pertinence, and stability and robustness, reducing the effect of low accuracy
- Summary
- Abstract
- Description
- Claims
- Application Information
AI Technical Summary
Problems solved by technology
Method used
Image
Examples
Embodiment 1
[0077] Embodiment 1: Experiment with the data of the SEED website without feature extraction, and the specific implementation steps are as follows.
[0078] Input: Contains 15*3 sample sets, each sample set contains 15 movie clips, each clip.
[0079] Output: The classification error rate on the test set.
[0080] (1) Definition: Given a set of 4 classifier algorithms {L 1 ,L 2 ,L 3 ,L 4}, where L 1 For the first learning algorithm - support vector machine (SVM) L 2 For the second learning algorithm - logistic regression classification (LR), L 3 For the third learning algorithm - K nearest neighbor algorithm (KNN). The sample set is defined as X, which means the EEG data of a person watching a movie clip on a certain day. The samples are divided into 1s time, and the label is defined as Y. X={X 1 ,X 2 ...X 2775}, Y={Y 1 ,Y 2 ...Y 2775}. where X i is the ith sample, Y i is the label of the ith sample. Sample X i ={x 1 (i) ,x 2 (i) ...x m (i)}, where x ...
Embodiment 2
[0113] Example 2: Experiment with the data extracted from the SEED website. The specific implementation is the same as that of Example 1.
[0114] Input: Contains 15*3 sample sets, each sample set contains 15 movie clips, each clip.
[0115] Output: The classification error rate on the test set.
[0116] It is verified by experiments that hierarchical bagging also has a good improvement effect on the data extracted by the SEED website, and the results are better than the single classifier algorithm and the traditional ensemble algorithm. Table 5 compares the results of the hierarchical Bagging algorithm on the feature-extracted data of the SEED website with the base classifier algorithm.
[0117] Table 5. Comparison of hierarchical Bagging algorithm with base classifier algorithm on SEED data after feature extraction
[0118]
[0119] Table 6 details the results of the hierarchical bagging algorithm on the feature-extracted data from the SEED website. Due to space const...
PUM
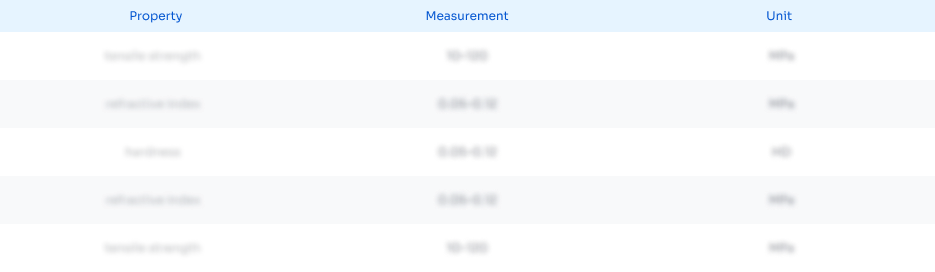
Abstract
Description
Claims
Application Information
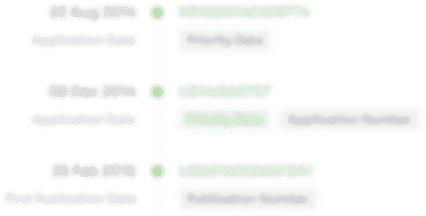
- R&D Engineer
- R&D Manager
- IP Professional
- Industry Leading Data Capabilities
- Powerful AI technology
- Patent DNA Extraction
Browse by: Latest US Patents, China's latest patents, Technical Efficacy Thesaurus, Application Domain, Technology Topic, Popular Technical Reports.
© 2024 PatSnap. All rights reserved.Legal|Privacy policy|Modern Slavery Act Transparency Statement|Sitemap|About US| Contact US: help@patsnap.com