Deep neural network multi-task hyper-parameter optimization method and device
A technology of deep neural network and optimization method, applied in the field of multi-task hyperparameter optimization of deep neural network, can solve the problem of large amount of calculation of hyperparameter optimization, and achieve the effect of speeding up learning and avoiding the amount of calculation.
- Summary
- Abstract
- Description
- Claims
- Application Information
AI Technical Summary
Problems solved by technology
Method used
Image
Examples
Embodiment 1
[0044] Aiming at the problem that the existing Bayesian optimization algorithm can only optimize a single task and cannot learn information between related tasks, the present invention proposes a multi-task learning network model (Multi-Task Learning, MTL) applied to Bayesian In the Yeesian optimization algorithm, multiple tasks are learned at the same time. Compared with the single-task learning method, each task can learn relevant information on other related tasks to promote its own learning and learn more feature information. In addition, the present invention also uses Radial Basic Function (RBF) neural network instead of the traditional Gaussian model for model training, which can reduce the amount of calculation and speed up the learning speed.
[0045] That is to say, the present invention connects the output of the radial basis neural network corresponding to multiple tasks through a fully connected layer, so that the information of multiple tasks can be shared, and th...
Embodiment 2
[0125] The present invention also provides an electronic device, which includes a memory, a processor, and a computer program stored on the memory and operable on the processing, when the processor executes the program, a deep neural network as described herein is implemented. Steps of a network multi-task hyperparameter optimization method.
Embodiment 3
[0127] The present invention also provides a computer-readable storage medium, on which a computer program is stored, and when the computer program is executed by a processor, the steps of a deep neural network multi-task hyperparameter optimization method as described herein are realized.
PUM
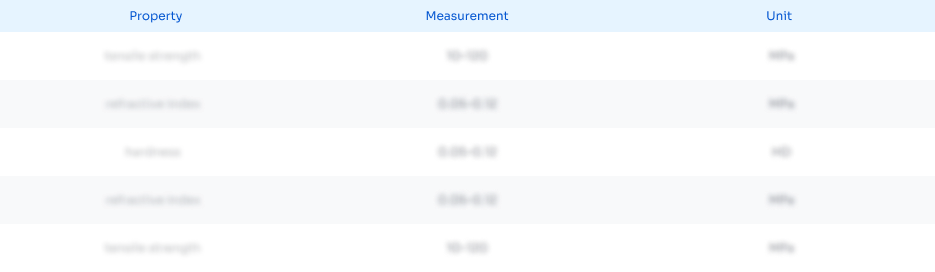
Abstract
Description
Claims
Application Information
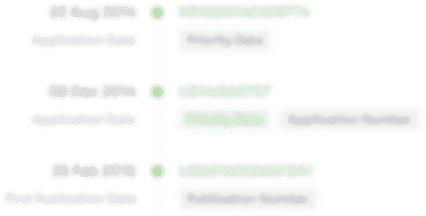
- R&D Engineer
- R&D Manager
- IP Professional
- Industry Leading Data Capabilities
- Powerful AI technology
- Patent DNA Extraction
Browse by: Latest US Patents, China's latest patents, Technical Efficacy Thesaurus, Application Domain, Technology Topic, Popular Technical Reports.
© 2024 PatSnap. All rights reserved.Legal|Privacy policy|Modern Slavery Act Transparency Statement|Sitemap|About US| Contact US: help@patsnap.com