Joint deep learning method capable of resisting generative adversarial network attacks
A deep learning and network attack technology, applied in the fields of privacy data protection and deep learning, can solve problems such as privacy destruction of deep learning model training data, and achieve the effect of strengthening initialization methods, preventing privacy leakage, and improving robustness
- Summary
- Abstract
- Description
- Claims
- Application Information
AI Technical Summary
Problems solved by technology
Method used
Image
Examples
Embodiment Construction
[0036] A joint deep learning method that can resist generational confrontational network attacks, and realize the system structure diagram of the present invention as shown in figure 1 As shown, four types of entities are included: Parameter Server (Parameter Server, PS), Blinding Server (Blinding Server, BS), Loyal User (Loyal User, LU), and Common Trainer (Common Trainer, CT). The parameter server PS is the initiator and scheduler of the entire joint learning task. It is responsible for initializing the joint learning system model, organizing and scheduling various entities to participate in joint learning according to the training process, and responsible for updating and distributing system model parameters during the joint learning process. It can be served by a cloud server that is semi-honest (curious about the private data of the trainer but does not initiate malicious attacks). The parameter server BS is a newly introduced third-party cloud server that does not collud...
PUM
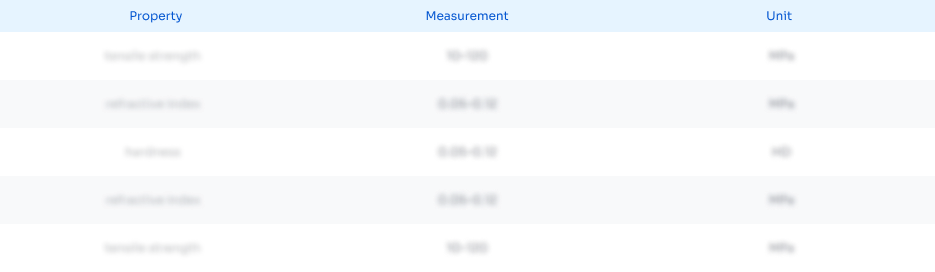
Abstract
Description
Claims
Application Information
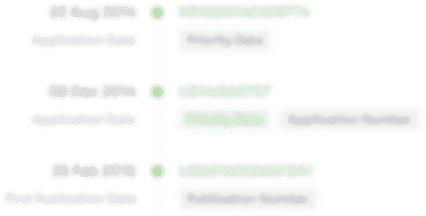
- R&D Engineer
- R&D Manager
- IP Professional
- Industry Leading Data Capabilities
- Powerful AI technology
- Patent DNA Extraction
Browse by: Latest US Patents, China's latest patents, Technical Efficacy Thesaurus, Application Domain, Technology Topic.
© 2024 PatSnap. All rights reserved.Legal|Privacy policy|Modern Slavery Act Transparency Statement|Sitemap