Multi-unmanned aerial vehicle 3D hovering position joint optimization method and device and unmanned aerial vehicle base station
A multi-UAV, joint optimization technology, applied in synchronization devices, vehicle position/route/altitude control, wireless communication, etc., can solve the problems of inability to apply to the actual communication environment, many limiting factors, and large gaps.
- Summary
- Abstract
- Description
- Claims
- Application Information
AI Technical Summary
Problems solved by technology
Method used
Image
Examples
Embodiment 1
[0061] Embodiment 1 of the present invention provides a joint optimization method for multi-UAV 3D hovering positions, such as figure 1 As shown, the method includes the following steps:
[0062] S101. Obtain status information of the heterogeneous network where the drone is located.
[0063] S102, input the state information into the pre-built deep reinforcement learning network, and decide the hovering position at the next moment through the current strategy.
[0064] A policy is a mapping from state to action.
[0065] S103, and obtain the reward function value of the hovering position of the drone at the current moment from the environment.
[0066] S104. Determine the gradient strategy algorithm based on the different strategy depth, and obtain an updated gradient.
[0067] S105, multiple UAVs synchronously update policy parameters.
[0068] S106, according to the gradient obtained in step S104, iteratively execute the steps from obtaining state information to synchro...
Embodiment 2
[0071] Embodiment 2 of the present invention provides another embodiment of a joint optimization method for 3D hovering positions of multiple UAVs.
[0072] The main flow chart of the optimization method provided by Embodiment 2 of the present invention is as follows figure 2 shown. The application scenario of the embodiment of the present invention is a heterogeneous network where a ground macro base station, a ground micro base station, and a UAV base station coexist. The ground communication terminal selects the base station for connection by judging the signal received power (RSRP). When the signal reception power of the adjacent base station meets the switching condition, the terminal switches the connected base station.
[0073] In this embodiment, first obtain the state information of the heterogeneous network environment, input the pre-established deep reinforcement learning network, the network uses the current policy function to determine the hovering position at t...
Embodiment 3
[0086] Embodiment 3 of the present invention provides another preferred embodiment of a joint optimization method for 3D hovering positions of multiple UAVs.
[0087] In the OPDPG algorithm, a different policy learning method is adopted, so the target policy obtained through training and the action policy of exploring the environment are different from each other. The target policy is a deterministic equation, in a given state s i next a i = π(s i ), for the UAV to greedily select the optimal action. However, the greedy algorithm cannot guarantee sufficient exploration and learning of the environment state, so the action strategy β(a|s) is introduced to take actions in the form of a random process for UAVs to explore unknown environments.
[0088] In the embodiment of the present invention, the OPDPG algorithm uses the action-evaluation method. The action-evaluation method combines value function-based and policy gradient-based reinforcement learning methods, inherits the ...
PUM
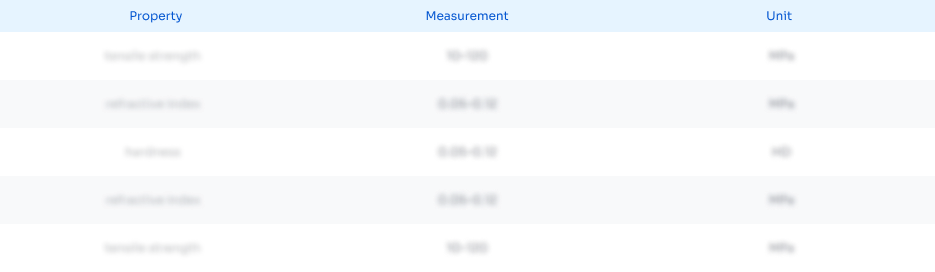
Abstract
Description
Claims
Application Information
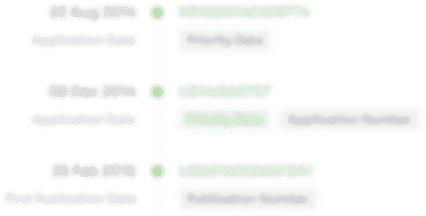
- R&D
- Intellectual Property
- Life Sciences
- Materials
- Tech Scout
- Unparalleled Data Quality
- Higher Quality Content
- 60% Fewer Hallucinations
Browse by: Latest US Patents, China's latest patents, Technical Efficacy Thesaurus, Application Domain, Technology Topic, Popular Technical Reports.
© 2025 PatSnap. All rights reserved.Legal|Privacy policy|Modern Slavery Act Transparency Statement|Sitemap|About US| Contact US: help@patsnap.com