Image defogging method based on global and local feature fusion
A local feature and feature map technology, applied in the field of image processing technology and deep learning, can solve the problems of artifacts and color distortion on the edge of objects, and achieve the effect of avoiding color distortion, no artifacts, and restoring image detail loss
- Summary
- Abstract
- Description
- Claims
- Application Information
AI Technical Summary
Problems solved by technology
Method used
Image
Examples
Embodiment 1
[0039] In order to achieve a realistic image defogging effect, an embodiment of the present invention proposes an image defogging method based on fusion of global and local features, see figure 1 , see the description below:
[0040] 101: Preprocessing the images in the training set;
[0041] 102: Construct a dehazing network based on the encoder-decoder architecture, set multiple densely connected units between the encoder and the decoder, and use the densely connected units to realize local and global fusion of feature maps;
[0042] 103: The feature map output by the encoder-decoder architecture passes through the subsequent convolutional neural network to obtain a dehazing map;
[0043] 104: Use the linear combination of L1 norm loss function, perceptual loss function and gradient loss function to train the dehazing network;
[0044] 105: After training, input a haze image to get a dehazed image.
[0045] Wherein, the specific steps of preprocessing the training set ima...
Embodiment 2
[0076] The scheme in Embodiment 1 is introduced in detail below in conjunction with specific drawings and calculation formulas, see the following description for details:
[0077] 201: Preprocessing the images in the training set;
[0078] 202: Construct a dehazing network based on the encoder-decoder architecture, set multiple densely connected units between the encoder and the decoder, and realize the local and global fusion of the feature map by the densely connected units;
[0079] 203: The feature map output by the encoder-decoder passes through the subsequent convolutional neural network to obtain a dehazing map;
[0080] 204: Use the linear combination of l1 norm loss function, perceptual loss function and gradient loss function to train the dehazing network;
[0081] 205: After training, input a haze image to obtain a dehazed image.
[0082] Wherein, the specific steps of preprocessing the training set images in step 201 are:
[0083] 1) The size of the pictures in ...
Embodiment 3
[0098] The scheme in embodiment 1 and 2 is carried out feasibility verification by experimental data below, see the following description for details:
[0099] Select 3 outdoor foggy images, use the defogging method of the present invention to remove the fog for these 3 foggy images, Figure 6 , Figure 7 and Figure 8 are the fogged image and the corresponding dehazed image, respectively.
[0100] It can be seen from the results of dehazing that the details covered by fog in the original image have been effectively restored, such as the details of windows in distant tall buildings become clearer after dehazing (such as Figure 6 shown); in addition, the brightness of the sky area in the defogged image changes naturally, and there are no halos, brightness, and contrast imbalances.
PUM
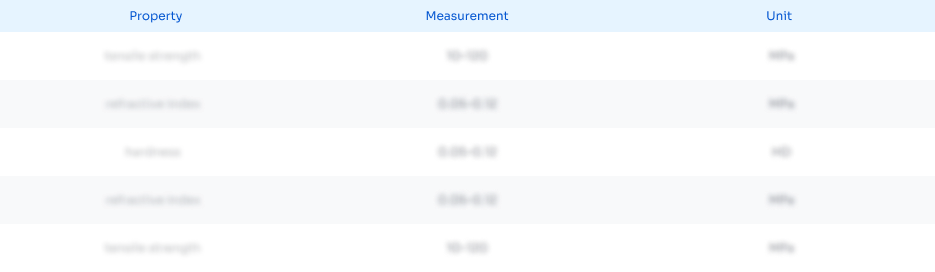
Abstract
Description
Claims
Application Information
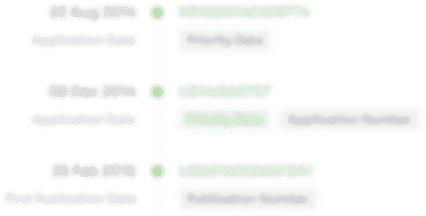
- R&D
- Intellectual Property
- Life Sciences
- Materials
- Tech Scout
- Unparalleled Data Quality
- Higher Quality Content
- 60% Fewer Hallucinations
Browse by: Latest US Patents, China's latest patents, Technical Efficacy Thesaurus, Application Domain, Technology Topic, Popular Technical Reports.
© 2025 PatSnap. All rights reserved.Legal|Privacy policy|Modern Slavery Act Transparency Statement|Sitemap|About US| Contact US: help@patsnap.com