Short-term residence load prediction method based on Attention-GRU
A load forecasting and residential technology, applied in forecasting, instrumentation, character and pattern recognition, etc., can solve the problems that RNN cannot guarantee the forecasting effect and is not suitable for dealing with long-term data dependence
- Summary
- Abstract
- Description
- Claims
- Application Information
AI Technical Summary
Problems solved by technology
Method used
Image
Examples
Embodiment Construction
[0036] The present invention will be further described in detail below in conjunction with the embodiments and the accompanying drawings, but the embodiments of the present invention are not limited thereto.
[0037] Such as Figure 1~2 As shown, a short-term residential load forecasting method based on Attention-GRU includes the following steps:
[0038] Step 1, data preprocessing;
[0039] The first step in load forecasting using a deep learning model is to use the appropriate format, e.g. LSTM and GRU models expect input 3D data, prepare the data with [samples, timesteps, features], and apply spatial clustering using density-based noise (DBSCAN ) technology to evaluate the consistency of daily power distribution; in the second step, construct training set and test set;
[0040]For each element of the data set and test set, there are two parts, namely matrix X and matrix Y; matrix X is the input of the gated recurrent neural network, and matrix Y is the output of the gated...
PUM
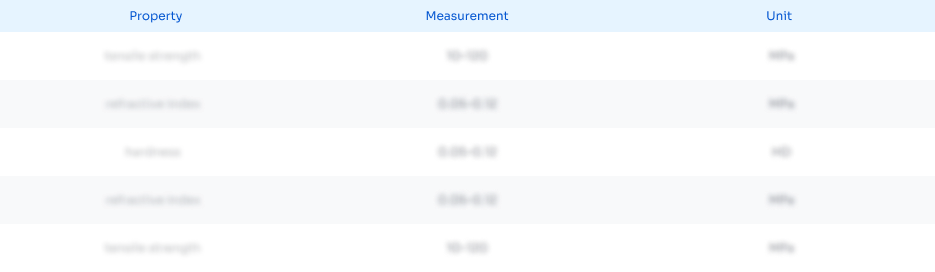
Abstract
Description
Claims
Application Information
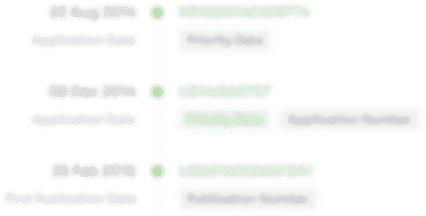
- R&D Engineer
- R&D Manager
- IP Professional
- Industry Leading Data Capabilities
- Powerful AI technology
- Patent DNA Extraction
Browse by: Latest US Patents, China's latest patents, Technical Efficacy Thesaurus, Application Domain, Technology Topic, Popular Technical Reports.
© 2024 PatSnap. All rights reserved.Legal|Privacy policy|Modern Slavery Act Transparency Statement|Sitemap|About US| Contact US: help@patsnap.com