Deep learning model-based traffic speed prediction method
A technology of deep learning and speed prediction, applied in neural learning methods, traffic flow detection, traffic control systems of road vehicles, etc., can solve problems such as limited decoder, difficulty in learning vector representation, and poor model performance
- Summary
- Abstract
- Description
- Claims
- Application Information
AI Technical Summary
Problems solved by technology
Method used
Image
Examples
experiment example
[0073] This experiment selects a publicly available large-scale traffic dataset - Q-Traffic dataset, which provides traffic speed data and various offline and online additional information. There are three kinds of additional information in the Q-Traffic dataset: 1) offline geographic and social information, including holidays, morning and evening peak hours, number of lanes, speed limit levels, etc.; 2) road network structure; 3) online map query information. The Q-Traffic dataset includes three sub-datasets in total: query sub-dataset, traffic speed sub-dataset and road network sub-dataset. The dataset is introduced as follows:
[0074] (1) Query sub-dataset
[0075] The query sub-dataset contains the map query information of Beijing from April 1, 2017 to May 31, 2017, which comes from Baidu Maps. Baidu Maps offers two map query modes: one called "Location Search," which includes searches for specific places, and another called "Route Search," which provides navigational r...
PUM
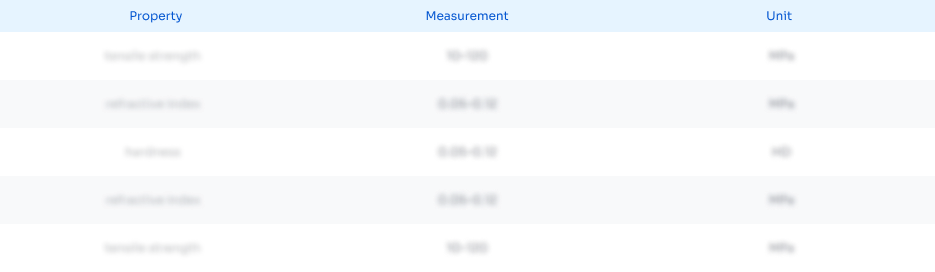
Abstract
Description
Claims
Application Information
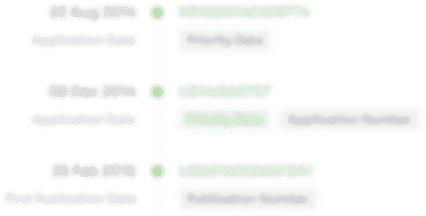
- R&D
- Intellectual Property
- Life Sciences
- Materials
- Tech Scout
- Unparalleled Data Quality
- Higher Quality Content
- 60% Fewer Hallucinations
Browse by: Latest US Patents, China's latest patents, Technical Efficacy Thesaurus, Application Domain, Technology Topic, Popular Technical Reports.
© 2025 PatSnap. All rights reserved.Legal|Privacy policy|Modern Slavery Act Transparency Statement|Sitemap|About US| Contact US: help@patsnap.com