Low-bit quantization method of depth separable convolution structure
A quantization method and low-bit technology, applied in neural learning methods, neural architectures, biological neural network models, etc., can solve problems such as network accuracy degradation, achieve the effects of reducing workload, facilitating implementation and application, and improving accuracy
- Summary
- Abstract
- Description
- Claims
- Application Information
AI Technical Summary
Problems solved by technology
Method used
Image
Examples
Embodiment Construction
[0046] In order to make the object, technical solution and advantages of the present invention clearer, the present invention will be described in further detail below in conjunction with specific embodiments and with reference to the accompanying drawings.
[0047] Such as figure 1 as shown, figure 1 It is a flowchart of a low-bit quantization method of a depth-separable convolution structure according to an embodiment of the present invention, and the method includes the following steps:
[0048] Step 1: Select the quantization coefficient to uniformly quantize the weight and feature map data of the depth separable convolutional neural network after training, so that the minimum mean square error of the weight and feature map data before and after quantization is the smallest;
[0049] Step 2: Quantize the weights in the deep separable convolutional neural network after training by channel;
[0050] Step 3: Input the picture, perform forward operation in the deep separab...
PUM
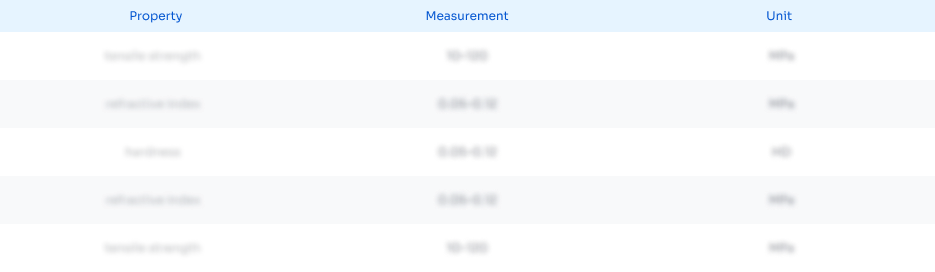
Abstract
Description
Claims
Application Information
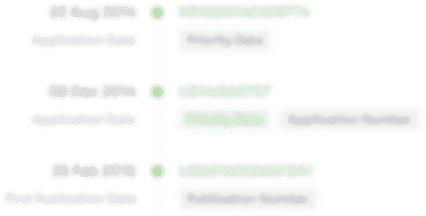
- R&D Engineer
- R&D Manager
- IP Professional
- Industry Leading Data Capabilities
- Powerful AI technology
- Patent DNA Extraction
Browse by: Latest US Patents, China's latest patents, Technical Efficacy Thesaurus, Application Domain, Technology Topic.
© 2024 PatSnap. All rights reserved.Legal|Privacy policy|Modern Slavery Act Transparency Statement|Sitemap