Four-rotor unmanned aerial vehicle route following control method based on deep reinforcement learning
A quadrotor UAV, reinforcement learning technology, applied in three-dimensional position/channel control, vehicle position/route/altitude control, attitude control and other directions, can solve the problem of unstable learning process, inability to achieve continuous control, low control accuracy, etc. question
- Summary
- Abstract
- Description
- Claims
- Application Information
AI Technical Summary
Problems solved by technology
Method used
Image
Examples
Embodiment
[0129]This implementation example realizes the autonomous flight of the quadrotor UAV to complete random route following. Set drone mass m=0.62 kg, gravity acceleration g=9.81 m / s 2 . Set the drone to be in the hovering state initially, and fly from the starting coordinates (0, 0, 0) to perform the task. When the drone finishes following the target route and reaches the end of the route, the system automatically refreshes the new target route, and the flight diagram of the drone performing the route following task is as follows: figure 2 shown.
[0130] The initial φ, θ, ψ are all 0°, which are derived from the identification of the UAV sensor. For the convenience of neural network processing, when the roll angle, pitch angle and yaw angle are input into the state, cosine processing is performed respectively. Set the drone's single-step movement time Δt = 0.05 seconds, and the quadrotor drone's thrust coefficient c T =0.00003, arm length d=0.23 meters.
[0131] Solve th...
PUM
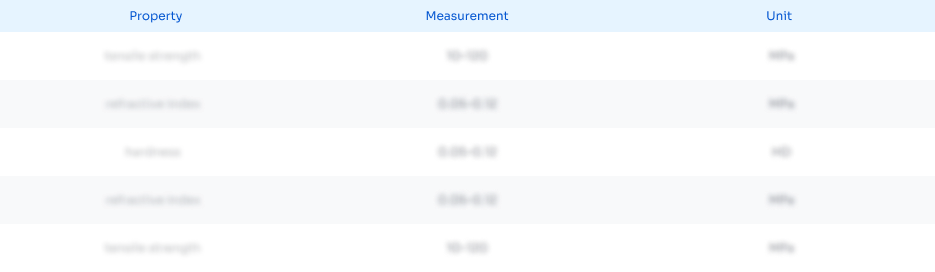
Abstract
Description
Claims
Application Information
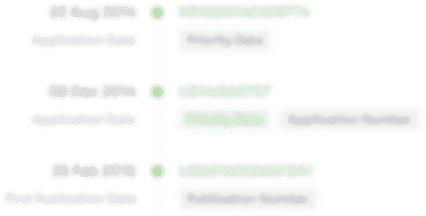
- R&D Engineer
- R&D Manager
- IP Professional
- Industry Leading Data Capabilities
- Powerful AI technology
- Patent DNA Extraction
Browse by: Latest US Patents, China's latest patents, Technical Efficacy Thesaurus, Application Domain, Technology Topic, Popular Technical Reports.
© 2024 PatSnap. All rights reserved.Legal|Privacy policy|Modern Slavery Act Transparency Statement|Sitemap|About US| Contact US: help@patsnap.com