A Data Center Fault Prediction Method Based on Data Augmentation
A fault prediction and data center technology, applied in neural learning methods, electrical digital data processing, generation of response errors, etc., can solve problems such as low accuracy, unbalanced training set, and poor results
- Summary
- Abstract
- Description
- Claims
- Application Information
AI Technical Summary
Problems solved by technology
Method used
Image
Examples
Embodiment Construction
[0044] The present invention provides a data center fault prediction method based on data enhancement. Aiming at the problem that the aforementioned data-driven node fault prediction technology receives less fault-related data and the unbalanced distribution of training set data results in a lower accuracy rate, a method is proposed. A data center fault prediction method based on data enhancement, that is, based on the combination of autoencoder and generative confrontation network, virtual data is generated by learning real data, thereby increasing the amount of fault-related data, and finally the fault is detected through LSTM network predict.
[0045] see figure 1 , the present invention a kind of data center fault prediction method based on data enhancement, comprises the following steps:
[0046] S1, data set preprocessing
[0047] First normalize the data points. Suppose the forecast horizon is t 1 , using the time point t and the previous R-1 data as the basis for p...
PUM
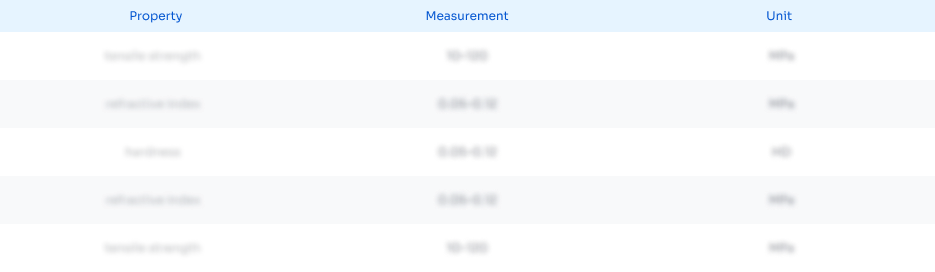
Abstract
Description
Claims
Application Information
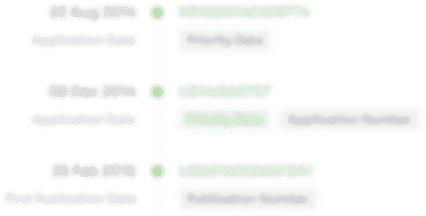
- R&D Engineer
- R&D Manager
- IP Professional
- Industry Leading Data Capabilities
- Powerful AI technology
- Patent DNA Extraction
Browse by: Latest US Patents, China's latest patents, Technical Efficacy Thesaurus, Application Domain, Technology Topic, Popular Technical Reports.
© 2024 PatSnap. All rights reserved.Legal|Privacy policy|Modern Slavery Act Transparency Statement|Sitemap|About US| Contact US: help@patsnap.com