Heterogeneous transfer learning method based on optimal subspace learning
A technology of subspace learning and transfer learning, applied in the field of heterogeneous transfer learning based on optimal subspace learning, which can solve the problems of heterogeneous transfer learning, differences in the distribution of source and target domains, and limited distribution.
- Summary
- Abstract
- Description
- Claims
- Application Information
AI Technical Summary
Problems solved by technology
Method used
Image
Examples
Embodiment Construction
[0067] The present invention will be further described in detail below in conjunction with the embodiments and the accompanying drawings, but the embodiments of the present invention are not limited thereto.
[0068] Such as figure 1 As shown, this embodiment is a heterogeneous transfer learning based on optimal subspace learning, and uses source domain images to classify target domain images. This embodiment includes the following steps:
[0069] S1: Obtain the source domain and target domain data for transfer learning from the database, and build a model with the objective function as the optimal subspace and classification learning based on typical correlation analysis methods. In this embodiment, the source domain samples and The target domain samples are all image data. The pictures themselves cannot be directly applied to algorithm learning. First, features need to be extracted from these samples. The number of samples in the source domain is sufficient, and image feat...
PUM
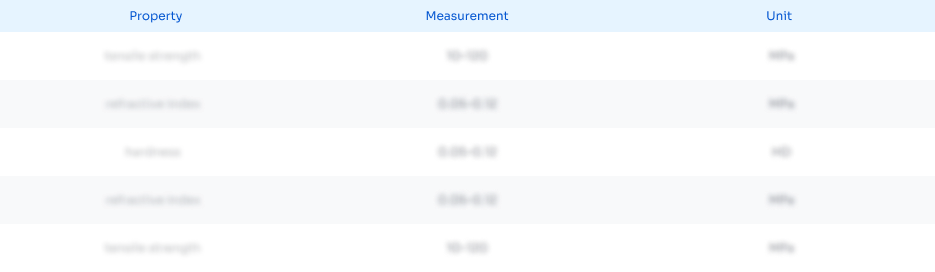
Abstract
Description
Claims
Application Information
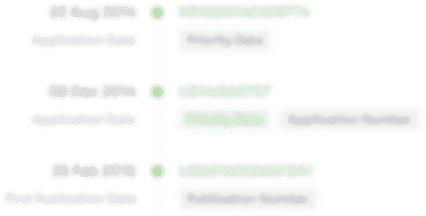
- R&D Engineer
- R&D Manager
- IP Professional
- Industry Leading Data Capabilities
- Powerful AI technology
- Patent DNA Extraction
Browse by: Latest US Patents, China's latest patents, Technical Efficacy Thesaurus, Application Domain, Technology Topic, Popular Technical Reports.
© 2024 PatSnap. All rights reserved.Legal|Privacy policy|Modern Slavery Act Transparency Statement|Sitemap|About US| Contact US: help@patsnap.com