A terminal access selection method based on deep reinforcement learning
A technology of reinforcement learning and terminal access, applied in the field of communication networks, to achieve the effects of improving transmission stability, improving resource utilization, and improving transmission rate
- Summary
- Abstract
- Description
- Claims
- Application Information
AI Technical Summary
Problems solved by technology
Method used
Image
Examples
Embodiment Construction
[0037] In order to facilitate those of ordinary skill in the art to understand the present invention, at first the following technical terms are defined:
[0038] 1. Q-Learning
[0039] A reinforcement learning algorithm, the agent perceives the environment by performing actions in the environment to obtain a certain reward, so as to learn the mapping strategy from state to action to maximize the reward value.
[0040] 2. Deep-Q-Learning (DQN)
[0041]DQN is the first to combine deep learning models with reinforcement learning to successfully learn control policies directly from high-dimensional inputs. By introducing the method of expected delayed return, the MDP (Markov Decision Process) problem under the condition of lack of information is solved. It can be considered that DQN learning is based on the instantaneous strategy and is a special deep reinforcement learning method of an independent model.
[0042] 3. Adaptive
[0043] According to the data characteristics of t...
PUM
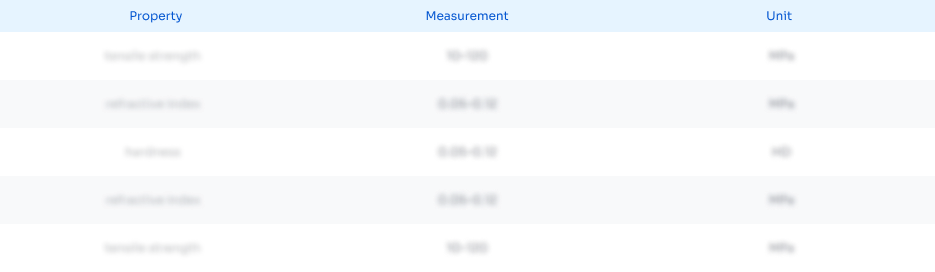
Abstract
Description
Claims
Application Information
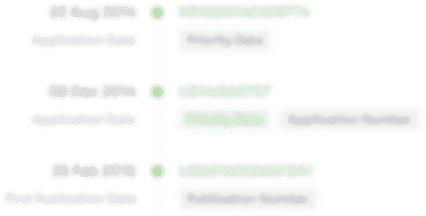
- R&D Engineer
- R&D Manager
- IP Professional
- Industry Leading Data Capabilities
- Powerful AI technology
- Patent DNA Extraction
Browse by: Latest US Patents, China's latest patents, Technical Efficacy Thesaurus, Application Domain, Technology Topic, Popular Technical Reports.
© 2024 PatSnap. All rights reserved.Legal|Privacy policy|Modern Slavery Act Transparency Statement|Sitemap|About US| Contact US: help@patsnap.com