Adaptive fault diagnosis method based on one-dimensional convolutional neural network
A convolutional neural network and fault diagnosis technology, which is applied in the fault diagnosis of parts and mechanical equipment, can solve problems such as loss, achieve the effect of small model, save diagnosis time, and save labor costs
- Summary
- Abstract
- Description
- Claims
- Application Information
AI Technical Summary
Problems solved by technology
Method used
Image
Examples
Embodiment Construction
[0026] The present invention will be further described in detail below with reference to the accompanying drawings and in combination with specific embodiments. It should be understood that the examples are only for illustration and not limitation of the present invention.
[0027] Such as figure 1 As shown, the present invention provides a kind of equipment, parts fault diagnosis method based on one-dimensional convolutional neural network, comprising the following steps:
[0028] Table 1 Rolling bearing fault type list
[0029]
[0030] Step 1. Collect the historical data of vibration acceleration signals under various operating states to diagnose faults through the acceleration sensor, and then perform the same adaptive filtering and normalization processing on the total sequence data of various faults to ensure the same distribution of data samples, and then Segment the total sequence data. In this embodiment, the faults are classified according to the different faul...
PUM
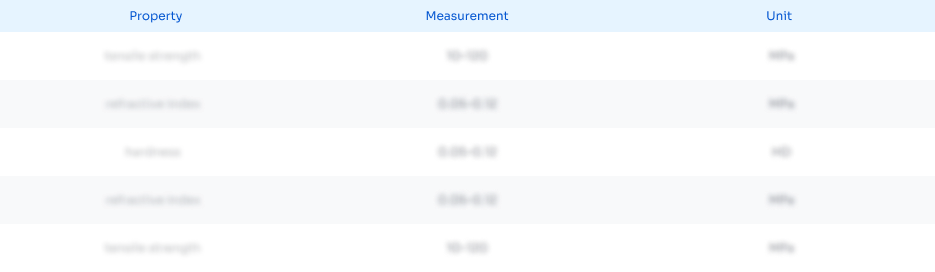
Abstract
Description
Claims
Application Information
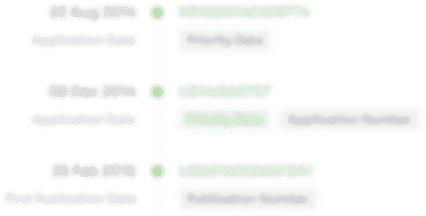
- R&D Engineer
- R&D Manager
- IP Professional
- Industry Leading Data Capabilities
- Powerful AI technology
- Patent DNA Extraction
Browse by: Latest US Patents, China's latest patents, Technical Efficacy Thesaurus, Application Domain, Technology Topic, Popular Technical Reports.
© 2024 PatSnap. All rights reserved.Legal|Privacy policy|Modern Slavery Act Transparency Statement|Sitemap|About US| Contact US: help@patsnap.com