Multi-dimensional time sequence abnormal value detection method and system based on MOTCN-AE
A MOTCN-AE, time series technology, applied in instruments, character and pattern recognition, computer parts, etc., can solve problems such as low accuracy, inability to meet the requirements of abnormal data detection accuracy, and inability to apply semi-supervised learning, etc. To achieve the effect of excellent precision
- Summary
- Abstract
- Description
- Claims
- Application Information
AI Technical Summary
Problems solved by technology
Method used
Image
Examples
Embodiment 1
[0052]In one or more embodiments, a multidimensional time series outlier detection method based on MOTCN-AE is disclosed, referring to figure 2 , including the following procedures:
[0053] (1) Receive a set of signals in the form of time series;
[0054] (2) Enriching the time series with features;
[0055] (3) Use an autoencoder to reconstruct the time series after feature enrichment;
[0056] (4) Comparing the feature-enriched time series with the reconstructed time series to obtain abnormal data in the time series form signal.
[0057] The method of this embodiment first applies temporal convolutional networks to the field of anomaly detection, and proposes a new time series anomaly detection framework MOTCN-AE (Multidimensional time series outlier detection based onTCN-AE) in combination with autoencoders and rich time series data. The framework consists of three parts: First, a TCN-based autoencoder TCN-AE is proposed using an unsupervised method combined with a tem...
Embodiment 2
[0133] In one or more embodiments, a multidimensional time series outlier detection system based on MOTCN-AE is disclosed, including:
[0134] means for receiving a set of signals in time-series form;
[0135] means for feature enriching said time series;
[0136] Apparatus for reconstructing feature-enriched time series using an autoencoder;
[0137] A device for comparing the feature-enriched time series with the reconstructed time series to obtain abnormal data in the time series signal.
[0138] Since the device provided in this embodiment can be used to implement the time series anomaly detection method in Embodiment 1, the technical effect it can obtain can refer to the above method embodiment, and will not be repeated here.
[0139] Those of ordinary skill in the art know that all or part of the steps in the above method can be completed by program instruction related hardware, and the program can be stored in a computer-readable storage medium, such as ROM, RAM and o...
PUM
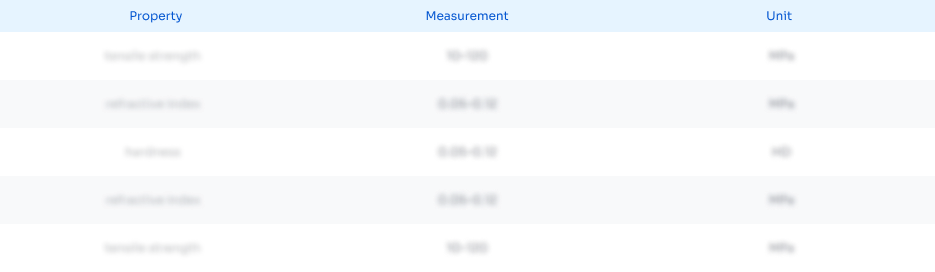
Abstract
Description
Claims
Application Information
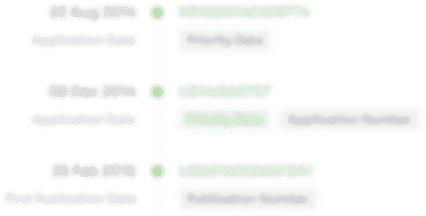
- R&D Engineer
- R&D Manager
- IP Professional
- Industry Leading Data Capabilities
- Powerful AI technology
- Patent DNA Extraction
Browse by: Latest US Patents, China's latest patents, Technical Efficacy Thesaurus, Application Domain, Technology Topic, Popular Technical Reports.
© 2024 PatSnap. All rights reserved.Legal|Privacy policy|Modern Slavery Act Transparency Statement|Sitemap|About US| Contact US: help@patsnap.com