Recurrent neural network-based remote sensing monitoring method and device for ground surface abnormal phenomenons
A cyclic neural network and abnormal phenomenon technology, which is applied in the field of remote sensing monitoring of surface anomalies based on cyclic neural network, can solve the problem that the robustness and applicability are not strong, it is difficult to find the change law and essential characteristics of the mathematical model, and the abnormal monitoring accuracy is not high. ideals etc.
- Summary
- Abstract
- Description
- Claims
- Application Information
AI Technical Summary
Problems solved by technology
Method used
Image
Examples
Embodiment Construction
[0049] The technical solution of the present invention will be further described below in conjunction with the accompanying drawings.
[0050] The schematic flow chart of the method for predicting surface anomalies based on the cyclic neural network provided in the embodiment of the present invention includes specific steps, which will be described in detail below in conjunction with the specific steps.
[0051] Step S101, obtaining the observation value of the observation location.
[0052] In the specific implementation, the observation location is the target location that needs to be observed and predicted; the observation value is usually expressed as the historical remote sensing image data of the observation location. Images can be acquired by remote sensing satellites according to the same revisit cycle, or by other image acquisition methods according to a certain time rule.
[0053] Step S102, forming a historical sequence of the observed values of the observed loca...
PUM
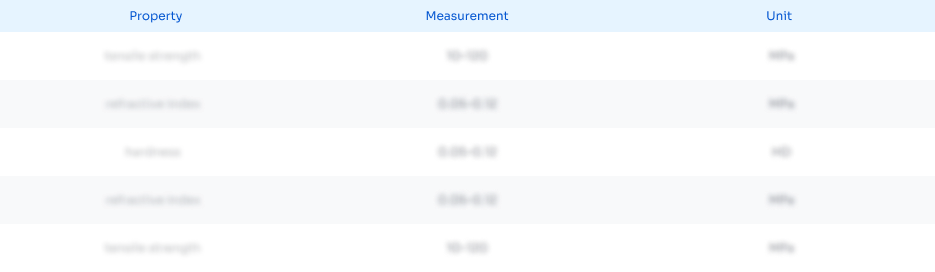
Abstract
Description
Claims
Application Information
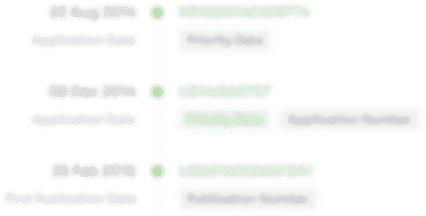
- R&D Engineer
- R&D Manager
- IP Professional
- Industry Leading Data Capabilities
- Powerful AI technology
- Patent DNA Extraction
Browse by: Latest US Patents, China's latest patents, Technical Efficacy Thesaurus, Application Domain, Technology Topic.
© 2024 PatSnap. All rights reserved.Legal|Privacy policy|Modern Slavery Act Transparency Statement|Sitemap