Traffic jam degree multi-dimensional analysis method based on deep learning
A technology for traffic congestion and analysis methods, applied in the field of deep learning, which can solve problems such as robustness, slow detection speed, and low detection accuracy
- Summary
- Abstract
- Description
- Claims
- Application Information
AI Technical Summary
Problems solved by technology
Method used
Image
Examples
Embodiment Construction
[0072] In order to make the object, technical solution and advantages of the present invention clearer, the present invention will be further described in detail below in conjunction with the accompanying drawings and embodiments. It should be understood that the specific embodiments described here are only used to explain the present invention, and do not limit the protection scope of the present invention.
[0073] like Figure 1-3 As shown, a kind of deep learning-based multi-dimensional analysis method of traffic congestion degree provided in this embodiment includes the following steps:
[0074] S1, camera preset position setting and camera calibration.
[0075] Specifically, adjust the camera to the appropriate traffic congestion analysis position, and set the current camera position as the preset position, and then capture a frame of image of the camera video stream, and perform lane lines, interest areas, and congestion level analysis areas on it calibration;
[007...
PUM
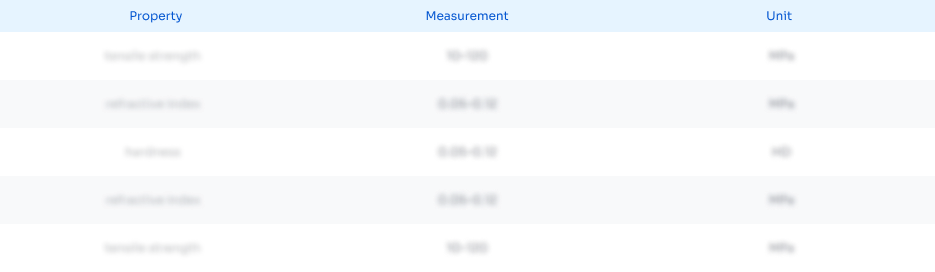
Abstract
Description
Claims
Application Information
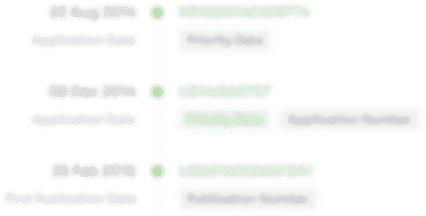
- R&D Engineer
- R&D Manager
- IP Professional
- Industry Leading Data Capabilities
- Powerful AI technology
- Patent DNA Extraction
Browse by: Latest US Patents, China's latest patents, Technical Efficacy Thesaurus, Application Domain, Technology Topic, Popular Technical Reports.
© 2024 PatSnap. All rights reserved.Legal|Privacy policy|Modern Slavery Act Transparency Statement|Sitemap|About US| Contact US: help@patsnap.com