Fault diagnosis method with noise label based on recurrent neural network
A cyclic neural network and fault diagnosis technology, applied in the direction of instruments, electrical testing/monitoring, control/regulation systems, etc., can solve problems such as low diagnostic accuracy
- Summary
- Abstract
- Description
- Claims
- Application Information
AI Technical Summary
Problems solved by technology
Method used
Image
Examples
Embodiment Construction
[0055] A fault diagnosis method with noise labels based on recurrent neural network, the method is realized by the following steps:
[0056] Step 1: Use the acceleration sensor to collect the vibration signals of the core components of the rotating machinery under different types of fault conditions, and normalize the vibration signals in sections to form a data set; the data set is expressed as:
[0057]
[0058]
[0059] the y m ∈{1,2,...,C};
[0060] In the formula: M represents the number of data samples in the data set; x m Indicates the mth data sample in the data set; y m Indicates the corresponding label of the mth data sample in the data set; Represents N-dimensional time series; {1,2,…,C} represents the type of fault;
[0061] Step 2: Generate the noise label of the data sample according to the corresponding label of the data sample; the noise label of the data sample is expressed as:
[0062]
[0063] In the formula: Indicates the noise label of the m...
PUM
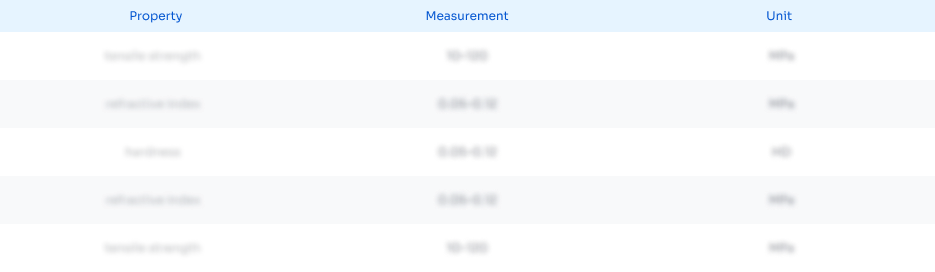
Abstract
Description
Claims
Application Information
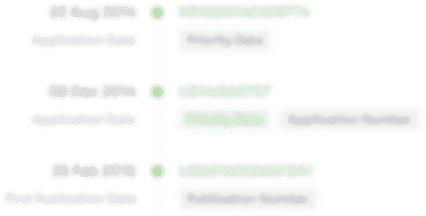
- R&D Engineer
- R&D Manager
- IP Professional
- Industry Leading Data Capabilities
- Powerful AI technology
- Patent DNA Extraction
Browse by: Latest US Patents, China's latest patents, Technical Efficacy Thesaurus, Application Domain, Technology Topic, Popular Technical Reports.
© 2024 PatSnap. All rights reserved.Legal|Privacy policy|Modern Slavery Act Transparency Statement|Sitemap|About US| Contact US: help@patsnap.com