State space probabilistic multi-time sequence prediction method based on graph neural network
A neural network and time series technology, applied in the field of state space probabilistic multi-time series prediction based on graph neural network, can solve problems such as poor generalization performance and difficult uncertainty estimation, and achieve the effect of avoiding complete correlation assumptions
- Summary
- Abstract
- Description
- Claims
- Application Information
AI Technical Summary
Problems solved by technology
Method used
Image
Examples
Embodiment Construction
[0020] In order to make the object, technical solution and advantages of the present invention clearer, the present invention will be further described in detail below in conjunction with the accompanying drawings and embodiments. It should be understood that the specific embodiments described here are only used to explain the present invention, and do not limit the protection scope of the present invention.
[0021] figure 1 It is the overall flowchart of the state space probabilistic multi-time series prediction method based on graph neural network provided by the embodiment. figure 2 It is the overall framework of the state space probabilistic multi-time series prediction method based on the graph neural network provided by the embodiment. figure 2 In the figure Z t Indicates the hidden state of each node at time t, X t Indicates the observed value of each node at time t, the solid arrow indicates the calculation of the generative model, and the dotted arrow indicates ...
PUM
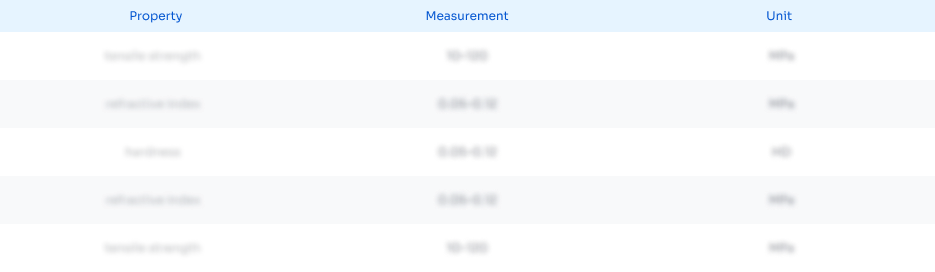
Abstract
Description
Claims
Application Information
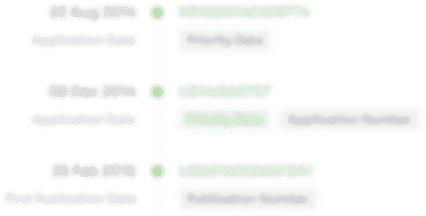
- R&D Engineer
- R&D Manager
- IP Professional
- Industry Leading Data Capabilities
- Powerful AI technology
- Patent DNA Extraction
Browse by: Latest US Patents, China's latest patents, Technical Efficacy Thesaurus, Application Domain, Technology Topic, Popular Technical Reports.
© 2024 PatSnap. All rights reserved.Legal|Privacy policy|Modern Slavery Act Transparency Statement|Sitemap|About US| Contact US: help@patsnap.com