Short-term power load prediction method based on similar days and RBF neural network
A short-term power load and neural network technology, applied to biological neural network models, predictions, neural architectures, etc., can solve problems such as no RBF model improvement, similar day selection has an impact, etc.
- Summary
- Abstract
- Description
- Claims
- Application Information
AI Technical Summary
Problems solved by technology
Method used
Image
Examples
Embodiment Construction
[0059] The present invention will be further described below in conjunction with the accompanying drawings and specific embodiments. The schematic embodiments and descriptions of the present invention are used to explain the present invention, but are not intended to limit the present invention.
[0060] A short-term power load forecasting method based on similar day and RBF neural network, comprising the following steps:
[0061] S1, quantify and normalize the influencing factors of electric load, the influencing factors include meteorological factors and date types; to establish a short-term power load forecasting model, firstly, analyze the relationship between load and influencing factors; meteorological factors and date types is the main influencing factor of the electric load. This embodiment mainly analyzes the impact of these two factors on the load. These load influencing factors have different dimensions, so they need to be processed before analyzing them together. Th...
PUM
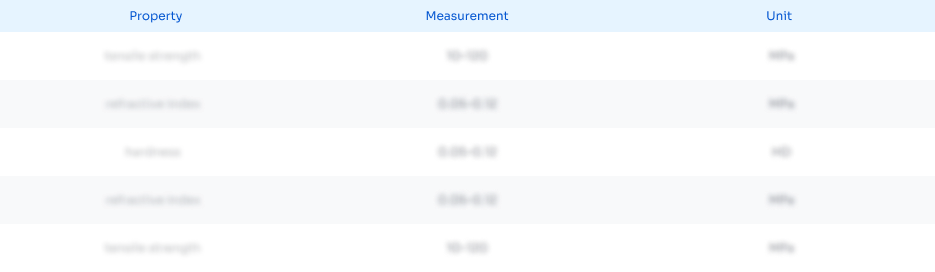
Abstract
Description
Claims
Application Information
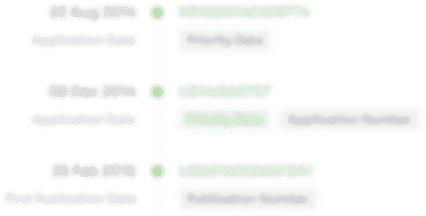
- R&D Engineer
- R&D Manager
- IP Professional
- Industry Leading Data Capabilities
- Powerful AI technology
- Patent DNA Extraction
Browse by: Latest US Patents, China's latest patents, Technical Efficacy Thesaurus, Application Domain, Technology Topic, Popular Technical Reports.
© 2024 PatSnap. All rights reserved.Legal|Privacy policy|Modern Slavery Act Transparency Statement|Sitemap|About US| Contact US: help@patsnap.com