Multi-region precipitation prediction model construction method based on multi-graph convolution and memory network
A technology for forecasting models and construction methods, which is applied in biological neural network models, forecasting, and neural learning methods. The effect of improving accuracy
- Summary
- Abstract
- Description
- Claims
- Application Information
AI Technical Summary
Problems solved by technology
Method used
Image
Examples
Embodiment Construction
[0050] The present invention will be further clearly and completely explained below in conjunction with the accompanying drawings and specific embodiments. Apparently, the described embodiments are only some of the embodiments of the present invention, not all of them.
[0051] figure 1 A multi-region precipitation prediction method based on multi-image convolution and memory network is provided, including the following:
[0052] (1) Making precipitation and environmental factors datasets
[0053] (1a) Collect the distance between M regions, the data of hydrological environmental factors, and the corresponding precipitation from each meteorological station, and remove the missing value and interference items.
[0054] (1b) In the multi-indicator evaluation system, due to the different properties of each evaluation index, it usually has different dimensions and orders of magnitude. When the levels of various indicators differ greatly, if the original indicator values are di...
PUM
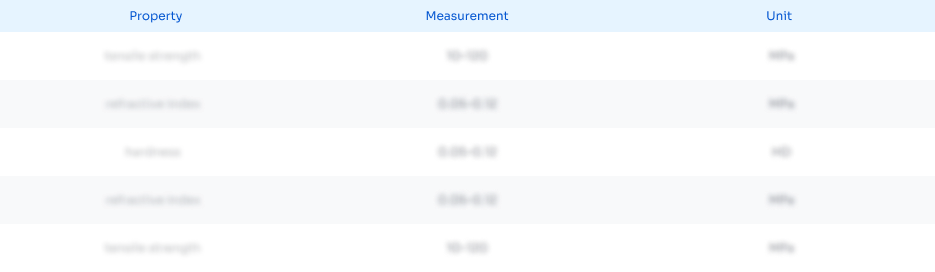
Abstract
Description
Claims
Application Information
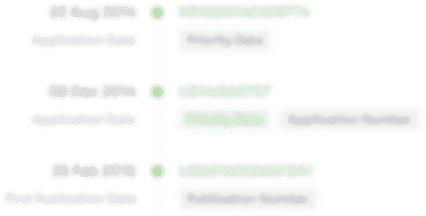
- R&D Engineer
- R&D Manager
- IP Professional
- Industry Leading Data Capabilities
- Powerful AI technology
- Patent DNA Extraction
Browse by: Latest US Patents, China's latest patents, Technical Efficacy Thesaurus, Application Domain, Technology Topic, Popular Technical Reports.
© 2024 PatSnap. All rights reserved.Legal|Privacy policy|Modern Slavery Act Transparency Statement|Sitemap|About US| Contact US: help@patsnap.com