Visual target tracking method of full-convolution integral type and regression twin network structure
A technology of target tracking and network structure, which is applied in the field of visual target tracking of full-volume classification and regression twin network structure, can solve the problem that the tracking effect is greatly affected, achieve good tracking accuracy, improve accuracy and speed, and have a simple network structure Effect
- Summary
- Abstract
- Description
- Claims
- Application Information
AI Technical Summary
Problems solved by technology
Method used
Image
Examples
Embodiment Construction
[0033] In order to make the present invention easier to understand and its advantages clearer, the technical solutions in the embodiments of the present invention will be described in detail below in conjunction with the drawings and specific embodiments.
[0034] refer to figure 1 and figure 2 , a visual target tracking method of full convolution classification and regression Siamese network structure, including the following steps:
[0035] (1) Select the visual target tracking training set, and cut out the target template image and the search area image in the original training set according to the position of the target in the image, and the cropped image pair constitutes the training data set;
[0036] (2) Build a fully convolutional twin network to extract image features. The fully convolutional twin network includes two branch networks with the same architecture, which are respectively a branch network for extracting target template image features and a branch network...
PUM
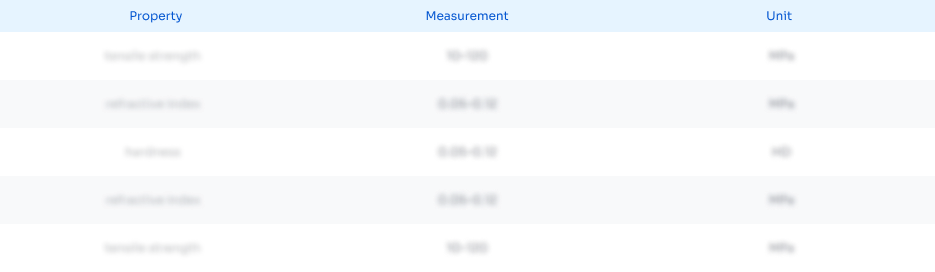
Abstract
Description
Claims
Application Information
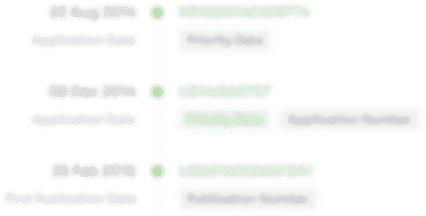
- R&D Engineer
- R&D Manager
- IP Professional
- Industry Leading Data Capabilities
- Powerful AI technology
- Patent DNA Extraction
Browse by: Latest US Patents, China's latest patents, Technical Efficacy Thesaurus, Application Domain, Technology Topic, Popular Technical Reports.
© 2024 PatSnap. All rights reserved.Legal|Privacy policy|Modern Slavery Act Transparency Statement|Sitemap|About US| Contact US: help@patsnap.com