Small sample SAR target identification method based on graph attention network
A technology of attention and small samples, applied in the field of image processing, can solve the problems of large amount of SAR image data, large similarity of training data, overfitting, etc., and achieve low computational complexity, improved recognition performance, and fast iteration speed Effect
- Summary
- Abstract
- Description
- Claims
- Application Information
AI Technical Summary
Problems solved by technology
Method used
Image
Examples
Embodiment Construction
[0026] Embodiments of the present invention will be further described below in conjunction with the accompanying drawings.
[0027] refer to figure 1 , the implementation steps of the present invention are as follows:
[0028] Step 1, constructing a data set for SAR small-sample recognition.
[0029] 1a) Select N SAR images containing radar targets from the MSTAR data set published on the Internet as a data set, and use a mean filter with a kernel size of 4×4 to suppress coherent speckle noise on all data, and obtain the SAR image after noise reduction;
[0030] 1b) Divide the denoised SAR image into labeled data and unlabeled data according to the ratio of 5% and 95%.
[0031] Step two, set up an autoencoder for extracting depth features of SAR images.
[0032] refer to figure 2 , the autoencoder consists of an encoder and a decoder, the input of the autoencoder is the denoised SAR image, and the output is the reconstructed SAR image;
[0033] The encoder consists of fi...
PUM
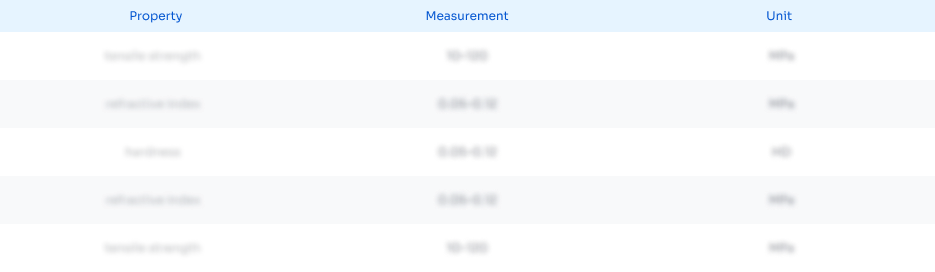
Abstract
Description
Claims
Application Information
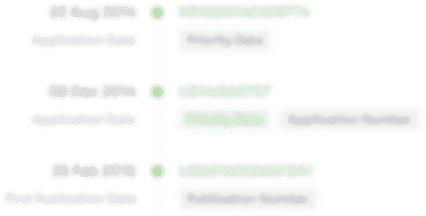
- R&D Engineer
- R&D Manager
- IP Professional
- Industry Leading Data Capabilities
- Powerful AI technology
- Patent DNA Extraction
Browse by: Latest US Patents, China's latest patents, Technical Efficacy Thesaurus, Application Domain, Technology Topic, Popular Technical Reports.
© 2024 PatSnap. All rights reserved.Legal|Privacy policy|Modern Slavery Act Transparency Statement|Sitemap|About US| Contact US: help@patsnap.com