Image super-resolution reconstruction method based on fused attention mechanism residual network
A super-resolution reconstruction and attention technology, applied in the field of image processing, can solve the problems of limited dictionary learning linear representation ability, slow convergence speed, lack of attention to high-frequency details, etc., to save training time and computing overhead, pixels Uniform distribution of data values, improved detail and clarity
- Summary
- Abstract
- Description
- Claims
- Application Information
AI Technical Summary
Problems solved by technology
Method used
Image
Examples
Embodiment Construction
[0058] The technical solution of the present invention is described in further detail below in conjunction with the accompanying drawings: the present embodiment is implemented on the premise of the technical solution of the present invention, and detailed implementation methods and specific operating procedures are provided, but the protection authority of the present invention does not Limited to the following examples.
[0059] This embodiment proposes an image super-resolution reconstruction method based on the fusion attention mechanism residual network, such as figure 1 shown, including the following steps:
[0060] S1. Data collection and preprocessing.
[0061] S11. Separately collect image data sets for training and image data sets to be reconstructed. In this embodiment, the standard public data set DIV2K is used as the training data set, including 800 training images, 100 verification images and 100 test images. The dataset to be reconstructed uses other standard ...
PUM
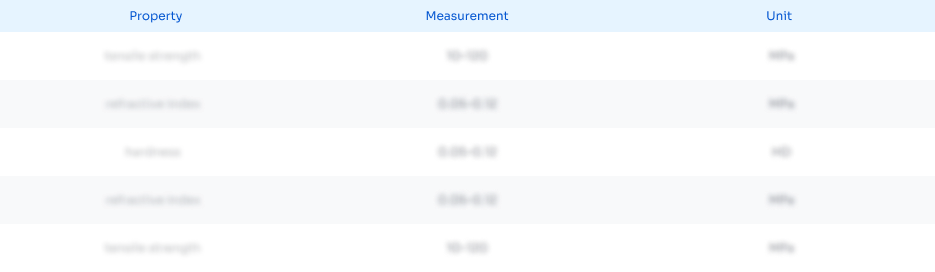
Abstract
Description
Claims
Application Information
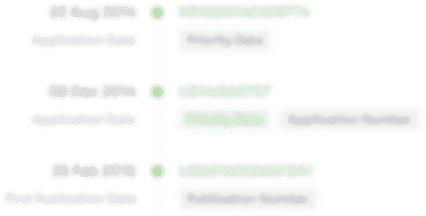
- R&D
- Intellectual Property
- Life Sciences
- Materials
- Tech Scout
- Unparalleled Data Quality
- Higher Quality Content
- 60% Fewer Hallucinations
Browse by: Latest US Patents, China's latest patents, Technical Efficacy Thesaurus, Application Domain, Technology Topic, Popular Technical Reports.
© 2025 PatSnap. All rights reserved.Legal|Privacy policy|Modern Slavery Act Transparency Statement|Sitemap|About US| Contact US: help@patsnap.com