Two-stage image retrieval method based on convolutional neural network
A convolutional neural network and image retrieval technology, which is applied in neural learning methods, still image data retrieval, biological neural network models, etc., can solve the problems of high computational cost and low search and matching efficiency, and achieve faster retrieval speed and improved query performance. efficiency effect
- Summary
- Abstract
- Description
- Claims
- Application Information
AI Technical Summary
Problems solved by technology
Method used
Image
Examples
Embodiment Construction
[0045] The following will clearly and completely describe the technical solutions in the embodiments of the present invention with reference to the accompanying drawings in the embodiments of the present invention. Obviously, the described embodiments are only some, not all, embodiments of the present invention. Based on the embodiments of the present invention, all other embodiments obtained by persons of ordinary skill in the art without making creative efforts belong to the protection scope of the present invention.
[0046] Such as figure 1 As shown, a two-stage image retrieval method based on convolutional neural network, the steps are as follows:
[0047] Step 1: Add a feature extraction layer between the convolutional layer and the densely connected layer of the VGG16 network to construct a convolutional neural network model with feature extraction and image classification capabilities; divide the data set into a training set, a verification set, and a test set.
[004...
PUM
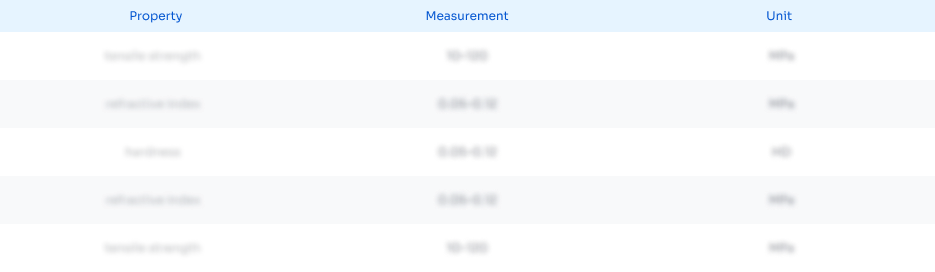
Abstract
Description
Claims
Application Information
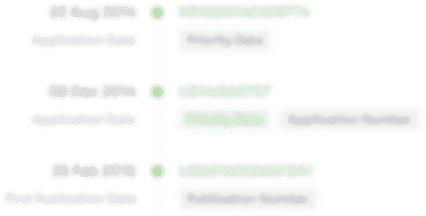
- R&D
- Intellectual Property
- Life Sciences
- Materials
- Tech Scout
- Unparalleled Data Quality
- Higher Quality Content
- 60% Fewer Hallucinations
Browse by: Latest US Patents, China's latest patents, Technical Efficacy Thesaurus, Application Domain, Technology Topic, Popular Technical Reports.
© 2025 PatSnap. All rights reserved.Legal|Privacy policy|Modern Slavery Act Transparency Statement|Sitemap|About US| Contact US: help@patsnap.com