Behavior identification method based on space-time context association
A technology of spatio-temporal context and recognition method, which is applied in the fields of deep learning, pattern classification and recognition, can solve problems such as unsatisfactory long-term correlation feature extraction performance, difficulty in applying battery capacity to wearable devices, and damage to the front and rear dependence characteristics of time series data, etc., to achieve The effect of increasing recognition accuracy, reducing loss, and increasing speed
- Summary
- Abstract
- Description
- Claims
- Application Information
AI Technical Summary
Problems solved by technology
Method used
Image
Examples
Embodiment Construction
[0027] In order to describe the technical content, structural features, achieved goals and effects of the present invention in detail, it will be described in detail in conjunction with the implementation modes and accompanying drawings.
[0028] The present invention proposes a behavior recognition method based on a deep network model based on spatio-temporal context features, which achieves good results in human behavior recognition. The schematic diagram of the whole algorithm is shown in figure 1 Shown, including training phases and steps:
[0029] The training steps include:
[0030] Step A1: Import the sensor perception data X of user behavior into the deep network model to perform convolution mapping operation to obtain convolution mapping data;
[0031] The overall user behavior of the above technical solutions is mainly divided into periodic behavior and sporadic behavior;
[0032] Periodic behavior: such as walking, running, cycling, etc.;
[0033] Scattered beha...
PUM
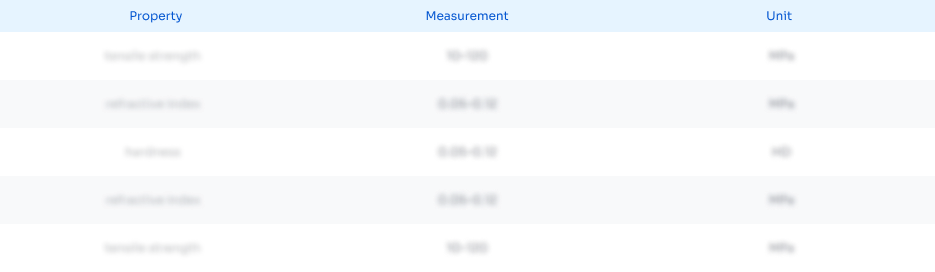
Abstract
Description
Claims
Application Information
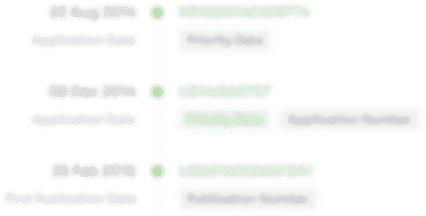
- R&D Engineer
- R&D Manager
- IP Professional
- Industry Leading Data Capabilities
- Powerful AI technology
- Patent DNA Extraction
Browse by: Latest US Patents, China's latest patents, Technical Efficacy Thesaurus, Application Domain, Technology Topic.
© 2024 PatSnap. All rights reserved.Legal|Privacy policy|Modern Slavery Act Transparency Statement|Sitemap