Malicious software classification method based on Markov graph and deep learning
A malware and deep learning technology, applied in the field of malware classification and deep learning, can solve the problems of difficult to quickly process a large number of malicious samples and low accuracy, and achieve high accuracy, reduce redundancy, and high classification accuracy Effect
- Summary
- Abstract
- Description
- Claims
- Application Information
AI Technical Summary
Problems solved by technology
Method used
Image
Examples
Embodiment Construction
[0026] The present invention will be described in further detail below in conjunction with the accompanying drawings and specific embodiments. Such as figure 1 Shown, a kind of malicious software family classification method based on Markov graph and deep learning of the present invention comprises the following steps:
[0027] Step 1: Count Byte Frequency
[0028] Think of malware bytes as a byte stream with timing characteristics, each byte as a specific state, and regard this malware byte stream as a random process, namely byte i ,i∈{0,1,...,N-1}, where N represents the number of malware bytes; traverse all the values of malware bytes, and count the frequency of occurrence of each value (256 types in total) .
[0029] Step 2: Calculate transition probabilities
[0030] Estimate the probability by frequency, and calculate the mutual transition probability between each state; if P i,j Indicates byte byte i Subsequent bytes are byte j The transition probability of , t...
PUM
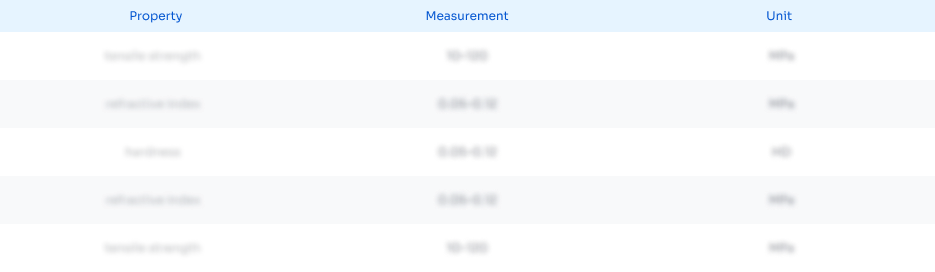
Abstract
Description
Claims
Application Information
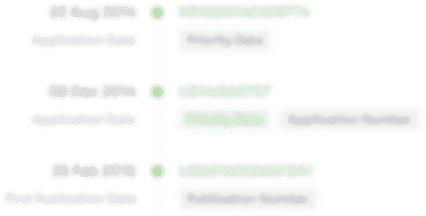
- R&D Engineer
- R&D Manager
- IP Professional
- Industry Leading Data Capabilities
- Powerful AI technology
- Patent DNA Extraction
Browse by: Latest US Patents, China's latest patents, Technical Efficacy Thesaurus, Application Domain, Technology Topic, Popular Technical Reports.
© 2024 PatSnap. All rights reserved.Legal|Privacy policy|Modern Slavery Act Transparency Statement|Sitemap|About US| Contact US: help@patsnap.com