Foreground object image segmentation method based on deep convolutional neural network
A neural network and deep convolution technology, applied in the field of computer vision, can solve the problems of decreased positioning accuracy, achieve high precision, strong generalization ability, and solve the effect of decreased positioning accuracy
- Summary
- Abstract
- Description
- Claims
- Application Information
AI Technical Summary
Problems solved by technology
Method used
Image
Examples
Embodiment Construction
[0032] In order to make the object, technical solution and advantages of the present invention clearer, the present invention will be further described in detail below in conjunction with the accompanying drawings and embodiments. It should be understood that the specific embodiments described here are only used to explain the present invention, not to limit the present invention.
[0033] The invention sets the segmentation task as a dense labeling problem, and proposes a pixel-level image segmentation model FOSeg model based on a deep convolutional neural network. The FOSeg model is a foreground object image segmentation model that supports end-to-end training and can predict the likelihood that each pixel is a foreground object.
[0034] The flow chart of FOSeg model segmentation is as follows: figure 1 As shown: first input the original image, after convolution, confluence, linear rectification and other operations of the deep convolutional neural network, and then input to...
PUM
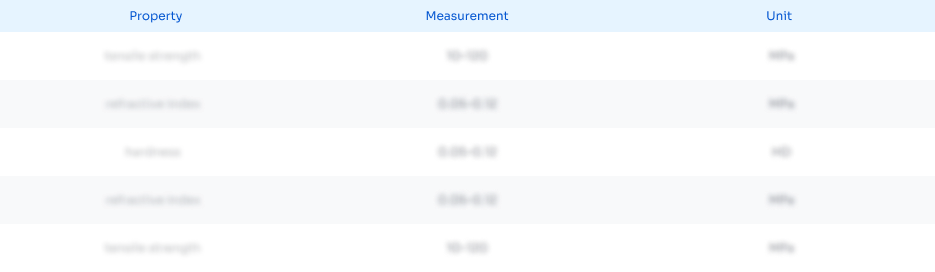
Abstract
Description
Claims
Application Information
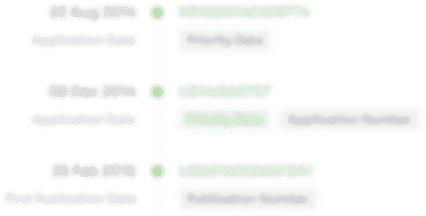
- R&D Engineer
- R&D Manager
- IP Professional
- Industry Leading Data Capabilities
- Powerful AI technology
- Patent DNA Extraction
Browse by: Latest US Patents, China's latest patents, Technical Efficacy Thesaurus, Application Domain, Technology Topic, Popular Technical Reports.
© 2024 PatSnap. All rights reserved.Legal|Privacy policy|Modern Slavery Act Transparency Statement|Sitemap|About US| Contact US: help@patsnap.com