An Approach to Attribute Reasoning and Product Recommendation Based on Adaptive Graph Convolutional Networks
A convolutional network and adaptive technology, applied in inference methods, neural learning methods, biological neural network models, etc., can solve the problem that the accuracy of attribute inference needs to be improved, so as to alleviate data sparsity, expand data dimensions, and improve The effect of precision
- Summary
- Abstract
- Description
- Claims
- Application Information
AI Technical Summary
Problems solved by technology
Method used
Image
Examples
Embodiment Construction
[0059] In this embodiment, an attribute reasoning product recommendation method based on an adaptive graph convolutional network considers the problem of lack of user and product attributes in the recommendation system, and performs feature propagation through an adaptive graph convolutional network to achieve more Precise attribute reasoning and product recommendation. Specifically, if figure 1 As shown, proceed as follows:
[0060] Step 1. Construct heterogeneous data, including: user rating matrix R for products, user attribute matrix X, product attribute matrix Y, user attribute index matrix A X , product attribute index matrix A Y :
[0061] Let U denote the user set, and U={u 1 ,...,u a ,...,u b ,...,u M},u a Indicates the ath user, u b Represents the bth user, M represents the total number of users, 1≤a, b≤M; let V represent the product set, and V={v 1 ,...,v i ,...,v j ,...,v N}, v i Indicates the i-th product, v j Represents the jth product, N represent...
PUM
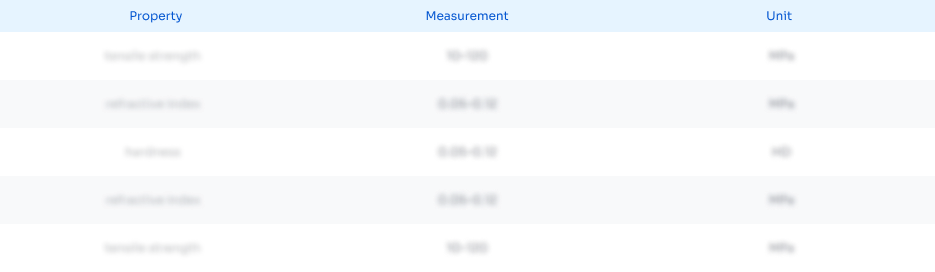
Abstract
Description
Claims
Application Information
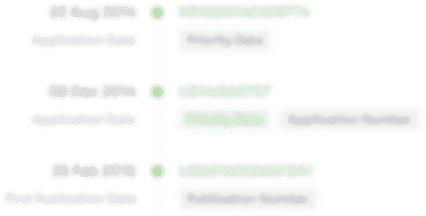
- R&D
- Intellectual Property
- Life Sciences
- Materials
- Tech Scout
- Unparalleled Data Quality
- Higher Quality Content
- 60% Fewer Hallucinations
Browse by: Latest US Patents, China's latest patents, Technical Efficacy Thesaurus, Application Domain, Technology Topic, Popular Technical Reports.
© 2025 PatSnap. All rights reserved.Legal|Privacy policy|Modern Slavery Act Transparency Statement|Sitemap|About US| Contact US: help@patsnap.com