Children epilepsy syndrome classification method based on transfer learning multi-model decision fusion
A technology of transfer learning and decision-making fusion, applied in the field of classification of epilepsy syndromes in children, can solve the problems of lack of early detection of epilepsy and intelligent auxiliary diagnosis system, limited medical resources, no intervention treatment and tracking evaluation system, etc.
- Summary
- Abstract
- Description
- Claims
- Application Information
AI Technical Summary
Problems solved by technology
Method used
Image
Examples
Embodiment Construction
[0067] Specific embodiments of the present invention will be described in detail below in conjunction with the accompanying drawings.
[0068] The first main step of the present invention is unsupervised artifact recognition, and its specific implementation steps are as follows:
[0069] 1-1 Do 0.5-70HZ bandpass filter and 50HZ notch filter for EEG signal.
[0070] 1-2 Divide the EEG signal into several 1-second periods, and perform clustering on the distance measure of Riemannian geometry through the covariance matrix to form several clusters and obtain the threshold.
[0071] 1-3 Divide the EEG signal into several 1-second periods, and the two periods overlap by 0.9 seconds. According to the covariance matrix and the centroid distances of several clusters formed by 1-2, the Z score is obtained.
[0072] 1-4 Use the Gaussian cumulative integral distribution function to convert the obtained Z score into a probability value, and then use the moving average filter to smooth the...
PUM
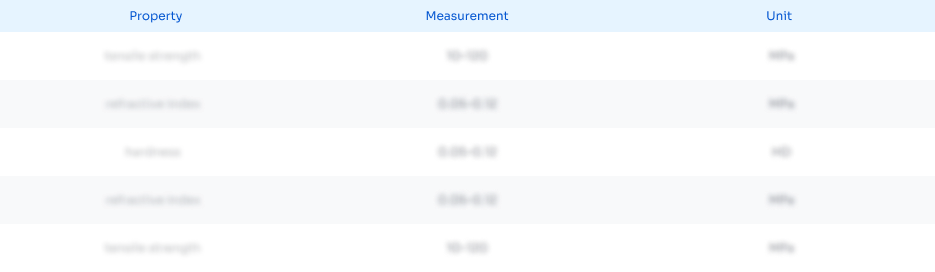
Abstract
Description
Claims
Application Information
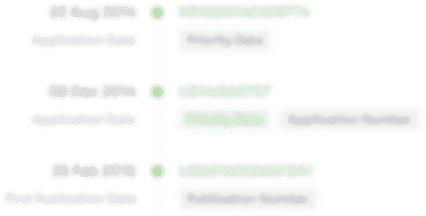
- R&D
- Intellectual Property
- Life Sciences
- Materials
- Tech Scout
- Unparalleled Data Quality
- Higher Quality Content
- 60% Fewer Hallucinations
Browse by: Latest US Patents, China's latest patents, Technical Efficacy Thesaurus, Application Domain, Technology Topic, Popular Technical Reports.
© 2025 PatSnap. All rights reserved.Legal|Privacy policy|Modern Slavery Act Transparency Statement|Sitemap|About US| Contact US: help@patsnap.com